Unlocking the Significance:
Understanding the Nuances of P-values
In the intricate dance of statistical analysis, the elusive p-value holds a position of paramount importance. It’s the litmus test, the gatekeeper, the arbiter of significance in the realm of research. Yet, amidst its widespread use, there exists a labyrinth of misunderstanding and misinterpretation. So, when is a p-value truly significant? Let’s embark on a journey through the corridors of statistical significance, unraveling its mysteries and shedding light on its nuanced nature.
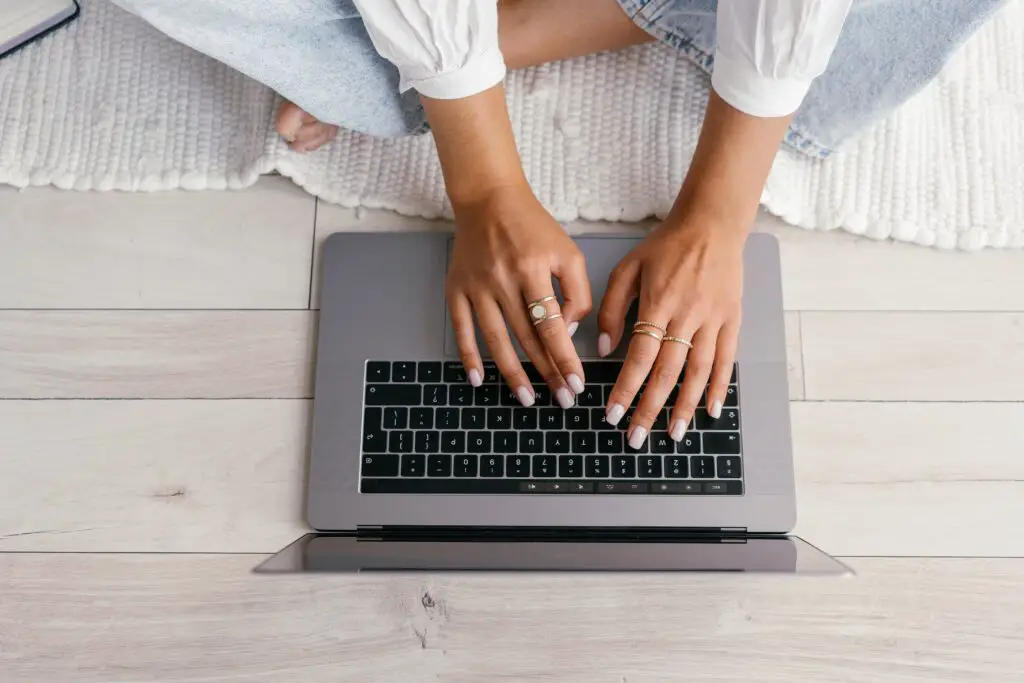
Navigating the Statistical Landscape
Before delving into the heart of the matter, it’s crucial to set the stage. Statistical analysis is the backbone of scientific inquiry, providing a systematic framework for drawing conclusions from data. At its core lies hypothesis testing, a methodological approach aimed at discerning whether observed differences are meaningful or merely due to chance.
Central to hypothesis testing is the notion of significance, quantified through the enigmatic p-value. This tiny numerical entity, often denoted as “p,” is the linchpin upon which decisions are made, hypotheses are accepted or rejected, and scientific narratives are crafted. But what does it truly signify? And when does it wield genuine significance?
When is P-value Significant?
Ah, the million-dollar question—when does the p-value assume significance? The answer, while seemingly straightforward, is cloaked in layers of complexity. A p-value is considered significant when it falls below a predetermined threshold, typically denoted as α (alpha), conventionally set at 0.05.
At this threshold, the p-value signifies that the observed results are unlikely to have occurred by random chance alone, under the assumption that the null hypothesis—the default assumption of no effect or no difference—is true. In simpler terms, it suggests that the evidence against the null hypothesis is strong enough to warrant its rejection, paving the way for the acceptance of the alternative hypothesis.
However, it’s imperative to tread cautiously in the realm of significance. While a p-value below the magical 0.05 mark may seem like the golden ticket to scientific acclaim, it’s essential to recognize its limitations. The significance of a p-value does not imply the magnitude or practical relevance of an effect. It merely indicates the strength of evidence against the null hypothesis within the confines of the study’s design and sample size.
Moreover, the interpretation of significance hinges on various factors, including study power, effect size, and the multiplicity of comparisons. A low p-value in isolation does not guarantee substantive or replicable findings. It’s merely a piece of the statistical puzzle, a fragment of the broader narrative awaiting contextualization and critical scrutiny.
The Dance of Probability:
Embracing Uncertainty
In the intricate tapestry of statistical inference, uncertainty reigns supreme. The p-value, while a powerful tool, serves as a beacon amidst the fog of uncertainty, guiding researchers toward tentative conclusions. Yet, it’s crucial to embrace the inherent uncertainty embedded within statistical analysis.
The p-value, by its very nature, is probabilistic—a product of randomness and variability. As such, it offers insights into the likelihood of observing the data under the null hypothesis but does not provide definitive proof of causality or truth. It’s a reflection of the inherent unpredictability of the natural world, where certainty is a rare commodity, and skepticism a guiding principle.
Navigating the Gray Areas:
Beyond Binary Thinking
In the dichotomous realm of statistical significance, shades of gray abound. The conventional dichotomy of significant versus nonsignificant fails to capture the nuances inherent in hypothesis testing. While a p-value below the sacred threshold may signal statistical significance, it does not denote absolute truth or irrefutable evidence.
It’s essential to adopt a nuanced perspective, one that acknowledges the continuum of evidence and the interplay between statistical significance and practical significance. A p-value slightly above the threshold may still offer valuable insights, especially in the presence of robust effect sizes or converging lines of evidence from multiple studies.
Moreover, the interpretation of p-values should be complemented by effect size estimation, confidence intervals, and a consideration of study design and methodology. Embracing uncertainty and exercising caution in interpretation are hallmarks of scientific rigor, safeguarding against the pitfalls of overinterpretation and false conclusions.
Conclusion
In the labyrinthine landscape of statistical analysis, the p-value stands as a beacon of significance, illuminating the path toward scientific discovery. Yet, its interpretation requires a delicate balance of skepticism and discernment, navigating the gray areas between certainty and uncertainty.
So, when is a p-value truly significant? The answer lies not in blind adherence to arbitrary thresholds but in a nuanced understanding of context, methodology, and interpretation. As we continue to unravel the mysteries of statistical inference, let us tread with humility, embracing uncertainty and charting a course toward more rigorous and nuanced scientific inquiry.