Unraveling the Mysteries of Normality Tests
In the labyrinth of statistics lies a fascinating realm where numbers hold secrets, and patterns whisper truths. Among the many tools wielded by statisticians and data scientists, the “normality test” stands as a sentinel, guarding the gates to understanding the distribution of data. In this journey through the realms of statistics, we shall venture into the heart of normality tests, uncovering their significance, applications, and intricacies.
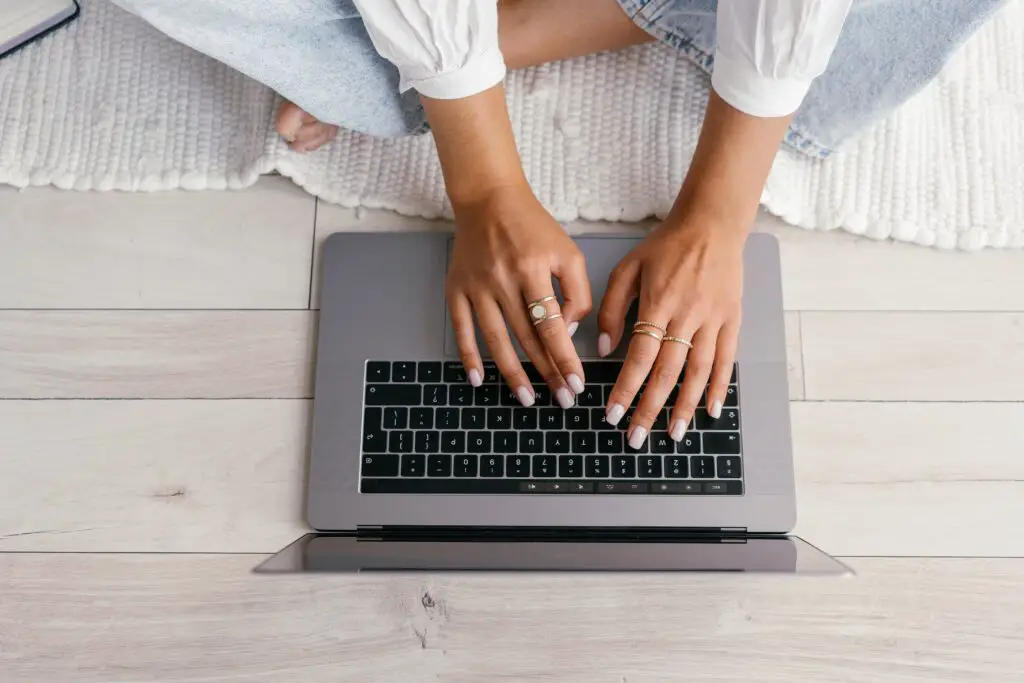
Normality Test:
Demystified
What is a Normality Test?
A normality test is a statistical procedure employed to determine if a dataset follows a normal distribution. But what exactly is a normal distribution? Picture a bell-shaped curve gracefully stretching across the axis, with its peak at the mean and its tails tapering off symmetrically. This idealized distribution, known as the Gaussian or bell curve, is the hallmark of normality.
Why Normality Matters?
The allure of normality lies in its ubiquity. Many statistical techniques, from parametric tests to regression analysis, assume or work best under the assumption of normality. Therefore, verifying whether our data conforms to this ideal distribution becomes paramount. A normality test acts as a detective, scrutinizing our data for deviations from this expected pattern.
Types of Normality Tests:
There exist various methodologies to assess normality, each with its own strengths and nuances. From the Shapiro-Wilk test, Anderson-Darling test, to Kolmogorov-Smirnov test, statisticians wield an arsenal of tools to probe the normality of data. Each test operates on different principles, catering to diverse scenarios and data characteristics.
Interpreting the Results:
Once the normality test has been conducted, the results beckon interpretation. If the p-value exceeds a predetermined threshold (often 0.05), we fail to reject the null hypothesis, indicating that our data is plausibly drawn from a normal distribution. Conversely, a p-value below the threshold warrants skepticism, suggesting departures from normality.
Challenges and Considerations:
However, interpreting normality tests isn’t always a straightforward affair. Sample size, skewness, and outliers can all confound our assessments. A large sample size might lead to statistical significance even for trivial deviations from normality, while outliers could unduly influence the results. Hence, caution and context remain our steadfast companions in the realm of statistics.
Applications in Practice:
Normality tests find their application in a myriad of fields, from finance to biology, from quality control to psychology. In finance, for instance, analysts may scrutinize the returns of assets to ascertain their distributional properties. In biology, researchers might assess the normality of experimental data to ensure the validity of statistical analyses. The applications are boundless, mirroring the omnipresence of data in our modern world.
The Evolving Landscape:
As data science burgeons and methodologies evolve, the landscape of normality testing continues to shift. New algorithms emerge, novel techniques surface, and debates ensue over the efficacy of traditional approaches. Amidst this flux, one thing remains certain—the quest for normality shall endure as long as data continues to weave the fabric of our understanding.
Conclusion:
In the tapestry of statistics, normality tests serve as crucial threads, weaving together the narrative of data distribution. From their humble beginnings as theoretical constructs to their indispensable role in modern analytics, these tests have weathered the tides of time. As we navigate the realms of data, let us not forget the humble yet mighty normality test, a beacon illuminating the path towards statistical enlightenment.