Sampling Error:
Navigating the Quirks of Data
In the realm of data analysis, the term “sampling error” is a beacon illuminating the often murky waters of statistical inference. It’s the phantom lurking in the shadows, ready to challenge the validity of our findings and inject a healthy dose of skepticism into our conclusions. But what exactly is this elusive concept, and how does it influence the reliability of our data? Let’s embark on a journey to unravel the mysteries of sampling error and understand its implications in the world of statistics.
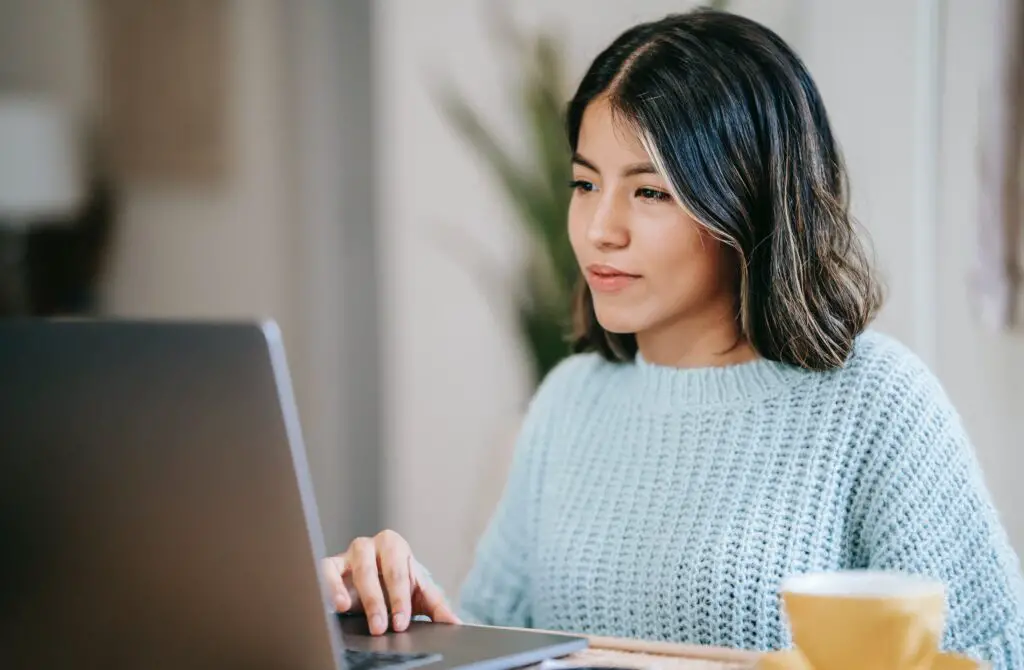
Sampling Error:
Unveiling the Enigma
Sampling error, in its essence, is the discrepancy between a sample statistic and the true population parameter it represents. Picture this: you’re at a bustling market, trying to estimate the average price of a kilogram of apples. Instead of weighing every single apple in the market (an impractical and exhausting task), you select a subset—a sample—of apples and calculate their average price. The difference between this sample mean and the true average price across all apples is the sampling error.
What Influences Sampling Error?
The magnitude of sampling error hinges on several factors, chief among them being sample size and variability within the population. A larger sample size generally leads to a smaller sampling error since it better represents the population’s characteristics. Conversely, a smaller sample size increases the likelihood of sampling error, as it may not accurately reflect the population’s diversity.
Variability within the population is another critical determinant of sampling error. If the population is highly homogeneous, meaning its members are very similar to each other, even a small sample can provide a reliable estimate of the population parameter. However, in a heterogeneous population with substantial differences among its members, a larger sample size is necessary to capture this diversity and minimize sampling error.
Mitigating Sampling Error:
Strategies and Solutions
While sampling error is an inherent challenge in statistical analysis, researchers employ various strategies to mitigate its impact and ensure the validity of their findings.
- Random Sampling: By randomly selecting samples from the population, researchers can minimize bias and increase the likelihood of obtaining a representative sample, thus reducing sampling error.
- Increasing Sample Size: As mentioned earlier, a larger sample size tends to result in a smaller sampling error. Researchers often strive to collect as much data as feasible within their constraints to enhance the reliability of their estimates.
- Stratified Sampling: In cases where the population exhibits significant heterogeneity, researchers may employ stratified sampling, dividing the population into distinct subgroups and then sampling from each subgroup proportionally. This approach ensures that all segments of the population are adequately represented, thereby reducing sampling error.
- Utilizing Confidence Intervals: Rather than relying solely on point estimates, researchers calculate confidence intervals around their sample statistics. These intervals provide a range of plausible values for the population parameter, accounting for the inherent uncertainty introduced by sampling error.
The Perils of Ignoring Sampling Error
Failing to account for sampling error can lead to flawed conclusions and misguided decision-making. Imagine a pharmaceutical company testing a new drug’s efficacy based on a small sample size. If they disregard sampling error and erroneously conclude that the drug is highly effective, they may proceed to mass production, only to discover later that the initial findings were misleading due to insufficient sampling. The consequences could be dire, jeopardizing both public health and financial investments.
In the realm of public opinion polling, sampling error plays a pivotal role in interpreting survey results. A political candidate leading in a poll may tout their popularity, but failing to acknowledge the margin of sampling error can lead to overconfidence and complacency. Similarly, a company launching a new product based on market research findings must account for sampling error to accurately gauge consumer preferences and mitigate potential risks.
Embracing Uncertainty:
A Humble Approach to Data Analysis
In a world inundated with data and metrics, it’s easy to fall prey to the allure of certainty. However, sampling error serves as a poignant reminder of the inherent uncertainty pervading statistical inference. Rather than viewing it as a hindrance, we should embrace it as a testament to the complexity of the world we seek to understand.
By acknowledging the presence of sampling error and adopting robust methodologies to address it, we can navigate the labyrinth of data with humility and integrity. In doing so, we not only enhance the reliability of our findings but also cultivate a deeper appreciation for the nuances of statistical analysis.
So the next time you encounter a statistical claim or research finding, pause for a moment and consider the specter of sampling error lurking beneath the surface. It may just be the key to unlocking a more nuanced understanding of the data-driven world we inhabit.