Unlocking the Secrets of Linear Regression:
Navigating the Assumptions
In the realm of statistical modeling, linear regression stands as a stalwart, a trusted tool employed to uncover relationships between variables and make predictions with a degree of certainty. However, beneath its seemingly straightforward facade lies a delicate ecosystem of assumptions. These assumptions, often overlooked or underestimated, serve as the bedrock upon which the efficacy and validity of linear regression rest.
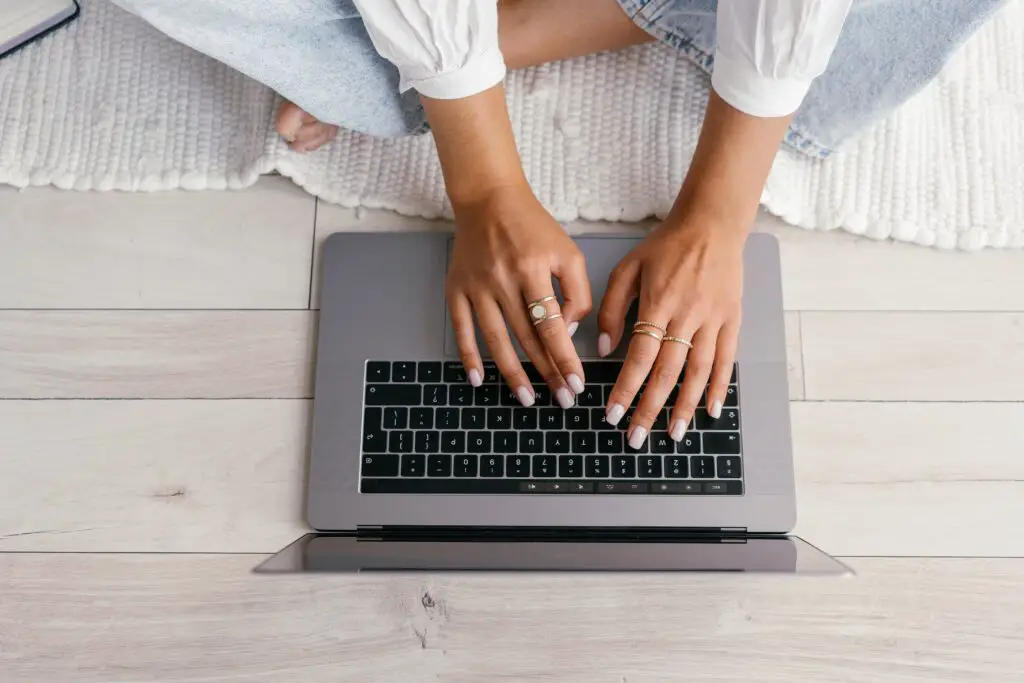
Assumptions in Linear Regression:
Unveiling the Core
Linear regression, with its simplicity and elegance, operates under a set of fundamental assumptions. Let’s delve into these assumptions to unravel the intricacies of this statistical technique:
1. Linearity
At the heart of linear regression lies the assumption of linearity, asserting that the relationship between the independent and dependent variables can be adequately captured by a straight line. This assumption posits that the change in the dependent variable is proportional to the change in the independent variable, holding true across the entire range of observations. However, in the real world, relationships between variables can often be more complex, necessitating careful scrutiny and potentially nonlinear transformations to adhere to this assumption.
2. Independence
Independence is another pillar upon which linear regression stands. This assumption posits that the observations or data points used in the regression analysis are independent of each other. In other words, the value of one observation should not be influenced by the values of other observations. Violation of this assumption, often encountered in time-series or spatial data, can lead to biased estimates and inflated standard errors, compromising the reliability of the regression results.
3. Homoscedasticity
Homoscedasticity, or the assumption of constant variance, asserts that the variability of the residuals, or the differences between the observed and predicted values, remains constant across all levels of the independent variables. In simpler terms, it implies that the spread of the data points around the regression line should be uniform. Departures from homoscedasticity, such as the presence of heteroscedasticity, can undermine the accuracy of the regression coefficients and render the standard errors invalid, casting doubt on the statistical inferences drawn from the model.
4. Normality of Residuals
The assumption of normality pertains to the distribution of the residuals, or the discrepancies between the observed and predicted values. Ideally, these residuals should follow a normal distribution, characterized by a symmetrical bell-shaped curve. Deviations from normality can arise due to outliers, skewness, or other non-normal patterns in the data. While violations of this assumption may not necessarily invalidate the regression results, they can affect the precision of confidence intervals and hypothesis tests, necessitating caution in interpretation.
5. No Multicollinearity
Multicollinearity refers to the presence of high correlations among the independent variables in the regression model. This assumption posits that each independent variable makes a unique and distinct contribution to the prediction of the dependent variable, without redundancy or overlap with other variables. Multicollinearity can inflate the variance of the regression coefficients, making them unstable and difficult to interpret. Techniques such as variance inflation factor (VIF) analysis are often employed to detect and mitigate multicollinearity in regression models.
6. No Autocorrelation
Autocorrelation, also known as serial correlation, occurs when the residuals of a regression model exhibit systematic patterns or correlations with themselves over time or space. This assumption is particularly relevant in time-series data, where observations are collected at regular intervals. Autocorrelation can distort the standard errors of the regression coefficients and lead to erroneous conclusions about the significance of the predictors. Diagnostic tests such as the Durbin-Watson statistic are employed to detect autocorrelation and guide appropriate model adjustments.
Navigating the Complexity
In the pursuit of knowledge and insight, linear regression serves as a guiding light, illuminating the relationships hidden within the labyrinth of data. Yet, as with any tool, its effectiveness hinges upon a thorough understanding of its assumptions and limitations. By acknowledging and scrutinizing these assumptions, researchers and practitioners can navigate the complexities of linear regression with confidence, unlocking its full potential as a vehicle for discovery and understanding in the realm of statistical analysis.