Exploring the Essence of Samples and Populations:
Unveiling the Heart of Statistical Analysis
In the realm of statistics, amidst the sea of data and numbers, lies a fundamental concept that serves as the bedrock of analysis: samples and populations. These seemingly simple terms harbor profound significance, shaping the way we understand and interpret data. In this article, we embark on a journey to unravel the essence of samples and populations, delving into their meanings, applications, and the intricate relationship that binds them.
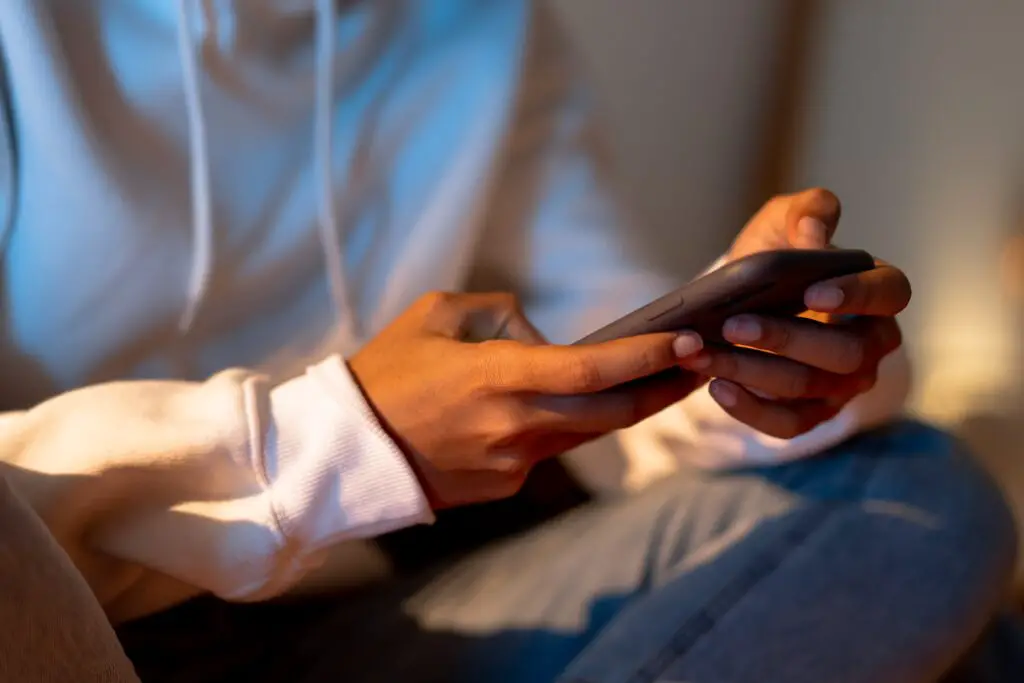
Sample and Population:
Understanding the Essence:
At the heart of statistical analysis lies the distinction between a sample and a population. Think of a population as the entirety of individuals, objects, or events that possess certain characteristics under consideration. It encompasses the entire group of interest, from which insights and conclusions are drawn. Conversely, a sample represents a subset of this population—a carefully selected segment that mirrors the larger whole. Imagine it as a miniature reflection, capturing the essence of the population while being more manageable for analysis.
Direct Answer:
Simply put, a sample is a subset of a population. It serves as a representative snapshot, allowing statisticians and researchers to draw conclusions about the larger group without having to examine every single element within it. Through the art of sampling, we extrapolate insights, make predictions, and infer characteristics of populations with confidence and precision.
Importance in Statistical Analysis:
The significance of samples and populations cannot be overstated in statistical analysis. They form the cornerstone upon which the edifice of inference is built. Consider a scenario where researchers aim to study the average income of households in a city. It’s impractical, if not impossible, to survey every household. Instead, they meticulously select a sample—a subset of households that mirrors the diversity and characteristics of the entire population. By analyzing this sample, they can draw conclusions about the population at large, with a certain degree of confidence and accuracy.
Types of Sampling Techniques:
Sampling techniques vary widely, each offering unique advantages and considerations. One common method is simple random sampling, where every member of the population has an equal chance of being selected. This approach ensures fairness and minimizes bias, making it a popular choice in many research endeavors. Alternatively, stratified sampling involves dividing the population into homogeneous subgroups or strata, and then selecting samples from each stratum. This technique ensures representation across different demographics or characteristics, offering insights into specific segments of the population.
Challenges and Considerations:
While sampling unlocks the door to understanding populations, it also presents challenges and considerations. Sampling bias, for instance, occurs when certain members of the population are systematically excluded or overrepresented in the sample. This can skew results and compromise the validity of conclusions drawn. Additionally, ensuring the representativeness and adequacy of a sample demands careful planning and methodology. Factors such as sample size, variability within the population, and sampling techniques must be meticulously considered to mitigate bias and ensure the reliability of results.
The Interplay with Statistical Inference:
Samples and populations dance in the realm of statistical inference, where insights are gleaned, hypotheses tested, and conclusions drawn. Through the lens of probability theory and inferential statistics, we navigate the intricacies of uncertainty, using samples as our guiding stars to illuminate the unknowns of populations. Whether estimating parameters, testing hypotheses, or making predictions, the relationship between samples and populations underpins the very fabric of statistical inference, guiding our quest for knowledge and understanding.
Conclusion:
In the tapestry of statistical analysis, samples and populations emerge as pivotal threads, weaving together the fabric of inference and understanding. They represent not merely abstract concepts, but the essence of our quest to unravel the mysteries hidden within data. Through meticulous sampling, careful analysis, and thoughtful interpretation, we unlock the secrets of populations, one sample at a time. As we continue to explore the realms of statistics and data science, let us remember the profound significance of samples and populations—the silent architects of insight and understanding in the ever-expanding universe of data.