Unveiling the Enigma:
Calculating Residuals in Regression Analysis
In the labyrinth of statistical analysis, where data reigns supreme and patterns hide in plain sight, lies the realm of regression analysis—a powerful tool wielded by researchers, analysts, and data enthusiasts alike. At its core, regression analysis endeavors to unravel the intricate relationships between variables, illuminating the path towards understanding and prediction. Yet, within this formidable framework, one aspect often mystifies even the most seasoned practitioners: calculating residuals.
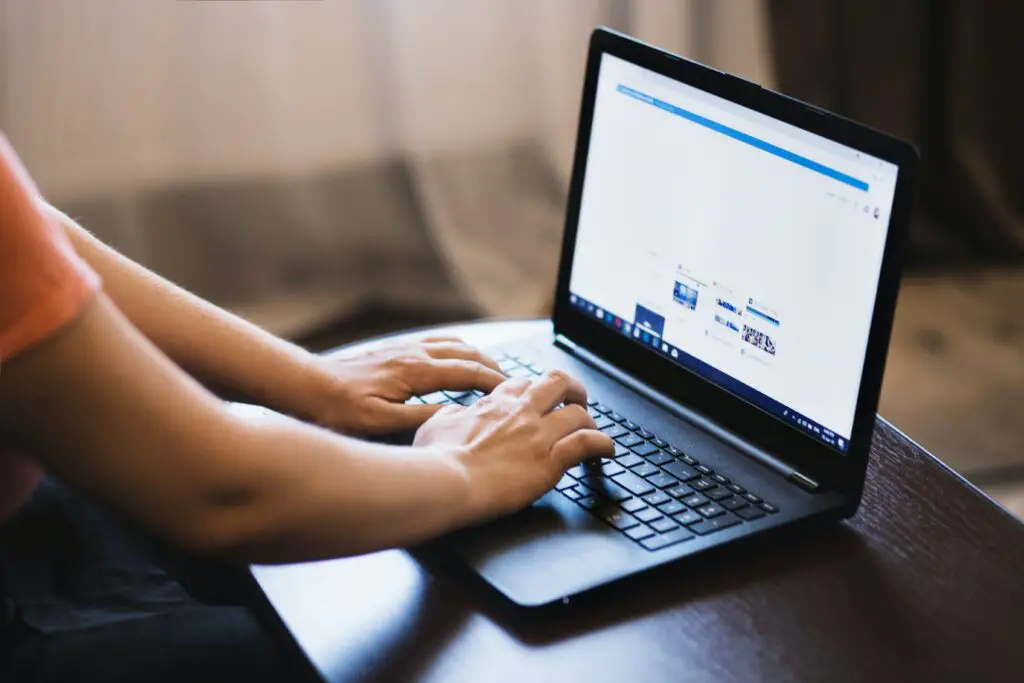
Exploring the Essence:
Calculating Residuals in Regression Analysis
Residuals, the unsung heroes of regression analysis, hold a pivotal role in assessing the fidelity of our models to reality. They embody the discrepancies between observed data points and their corresponding predictions—a measure of how well our model captures the nuances of the phenomenon under scrutiny.
To calculate residuals, we embark on a journey through the heart of our regression model. Imagine a scatterplot, where each point represents a data observation—a glimpse into the complex interplay of variables. Our regression line, a beacon of mathematical prowess, strives to weave through this constellation of points, minimizing the chasm between prediction and reality.
But alas, perfection eludes us. Residuals emerge as the residue of this endeavor, the remnants of our model’s attempt to encapsulate the essence of the data. To compute these residuals, we simply subtract the predicted value (as dictated by our regression equation) from the observed value for each data point.
In mathematical terms, the residual (ε) for each observation can be expressed as:
ε = Observed Value – Predicted Value
This elegant formula encapsulates the essence of residuals—the essence of capturing the nuances that evade our models’ grasp. A positive residual signifies an observed value surpassing our model’s prediction, while a negative residual denotes the opposite—a gap between expectation and reality.
Navigating the Terrain:
Practical Considerations
In the realm of regression analysis, understanding how to calculate residuals is merely the first step in a journey fraught with challenges and insights. Practical considerations abound, demanding a nuanced approach to interpretation and analysis.
Consider, for instance, the distribution of residuals—a window into the efficacy of our model. A symmetrical distribution, centered around zero, suggests that our model captures the essence of the data without bias. Conversely, skewed distributions may betray the presence of systematic errors or unaccounted variables, beckoning us to refine our approach.
Moreover, residuals serve as the cornerstone of diagnostic tests, enabling us to unearth hidden assumptions and model misspecifications. Scatterplots of residuals against predicted values or independent variables unveil patterns—patterns that hint at lurking complexities or nonlinear relationships yearning to be unraveled.
In the pursuit of knowledge, outliers stand as formidable adversaries, challenging the very foundations of our analysis. Residual plots, coupled with robust statistical techniques, empower us to identify and address these outliers, ensuring the integrity and robustness of our conclusions.
Embracing the Journey:
Conclusion
In the labyrinth of regression analysis, where data intertwines with theory, and insight illuminates the path forward, calculating residuals emerges as both an art and a science. It is a testament to our endeavor to unravel the mysteries of the universe, one data point at a time.
As we navigate the terrain of regression analysis, let us not overlook the significance of residuals—the whispers of truth amidst the cacophony of data. For in their subtlety lies the key to unlocking the secrets of our models and the phenomena they seek to elucidate.
So, let us embark on this journey with curiosity and humility, for in the realm of regression analysis, the quest for knowledge knows no bounds, and the mysteries that await us are as boundless as the human imagination itself.