Unlocking the Mysteries of Chi-Square Analysis
In the labyrinth of statistical analysis, one method stands out as both ubiquitous and enigmatic: the chi-square test. Its applications range from genetics to market research, from medicine to sociology. Yet, for many, it remains a puzzle wrapped in an enigma. Fear not, for we are about to embark on a journey through the intricacies of chi-square analysis, shedding light on its principles, applications, and nuances. So, buckle up and prepare to demystify the chi-square!
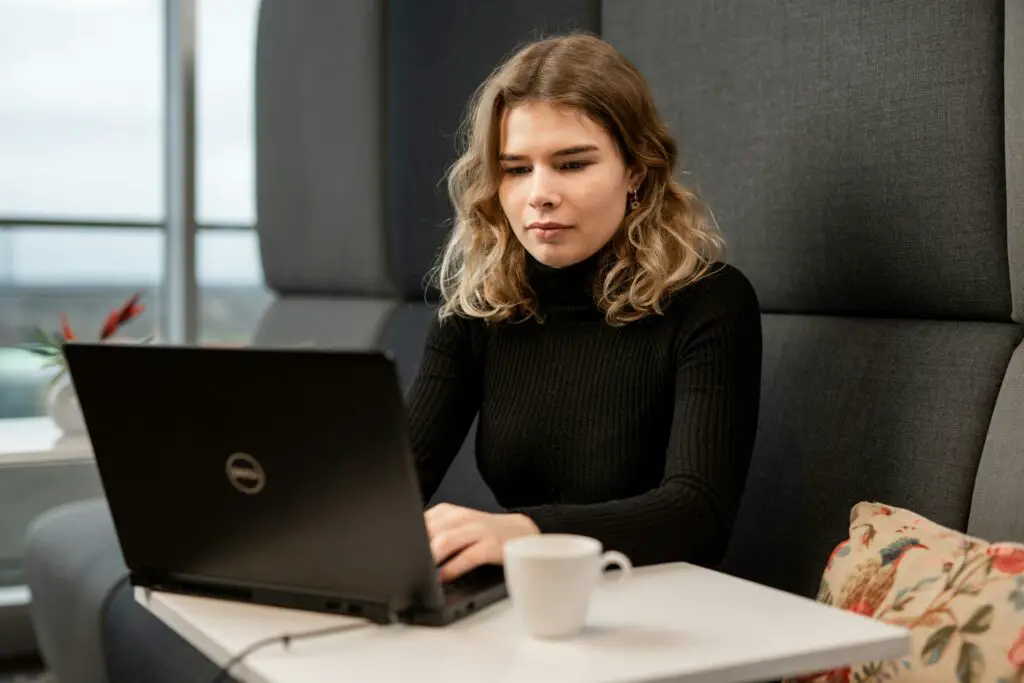
Report Chi-Square:
Unraveling the Mystery
Ah, the Chi-Square – a phrase that may evoke dread or curiosity in the hearts of many. But what exactly is it? In simplest terms, the chi-square test is a statistical method used to determine whether there is a significant association between categorical variables. This association could be in the form of independence, homogeneity, or goodness-of-fit.
What Does the Chi-Square Test Tell Us?
In essence, the chi-square test provides a means to evaluate whether observed frequencies of categorical data differ significantly from the frequencies we would expect if the variables were independent. Imagine tossing a coin and recording the outcomes – if the coin is fair, we’d expect roughly equal frequencies of heads and tails. The chi-square test helps us determine if the deviations from this expected distribution are due to chance or if there’s a genuine relationship between variables.
How Is Chi-Square Calculated?
The calculation of the chi-square statistic involves comparing observed frequencies with expected frequencies under the assumption of independence. This is typically done by creating a contingency table, also known as a cross-tabulation, where the rows represent one variable and the columns represent the other. The formula for the chi-square statistic involves summing up the squared differences between observed and expected frequencies, normalized by the expected frequencies.
Applications of Chi-Square Analysis
Now that we’ve grasped the basics, let’s explore the myriad applications of chi-square analysis. In biology, it’s used to study genetic inheritance patterns and assess whether observed ratios match expected Mendelian ratios. In social sciences, it helps researchers understand relationships between demographic variables, such as gender and voting preferences. In market research, it’s employed to analyze consumer preferences and buying behavior.
Chi-Square Variants
As with any statistical tool, the chi-square test comes in various flavors tailored to different scenarios. The most common variants include the Pearson chi-square test, used for goodness-of-fit analysis, and the likelihood ratio chi-square test, which is more robust for smaller sample sizes. There’s also Fisher’s exact test, suitable for contingency tables with small expected cell counts.
Interpreting the Results
Once the chi-square statistic is calculated, the next step is to assess its significance. This is typically done by comparing the calculated chi-square value to a critical value from the chi-square distribution with appropriate degrees of freedom. If the calculated chi-square value exceeds the critical value, we reject the null hypothesis and conclude that there is a significant association between the variables.
Common Pitfalls and Limitations
While powerful, the chi-square test is not without its limitations. One common pitfall is the assumption of independence between variables, which may not always hold true. Additionally, the test may be sensitive to sample size, leading to spurious results with small datasets. Care must also be taken with the interpretation of significant results, as correlation does not imply causation.
Conclusion
In our journey through the realm of chi-square analysis, we’ve peeled back the layers of complexity surrounding this fundamental statistical tool. From its humble beginnings to its diverse applications, the chi-square test remains a cornerstone of data analysis across disciplines. Armed with this newfound knowledge, may you venture forth with confidence into the world of categorical data, knowing that the chi-square is but a friend, waiting to unlock the secrets hidden within the numbers.