Exploring the Foundations of Chi-Square Analysis:
Unveiling Its Assumptions
In the intricate tapestry of statistical analysis, Chi-square emerges as a versatile tool, a beacon guiding researchers through the labyrinth of data exploration. Its utility spans across various fields, from biology to sociology, from psychology to economics. But like any sophisticated apparatus, Chi-square rests upon certain foundational assumptions, pillars upon which its efficacy stands tall. In this exploration, we delve into the nuanced assumptions underpinning Chi-square analysis, unraveling the essence of its application and ensuring its robustness in extracting meaningful insights from data.
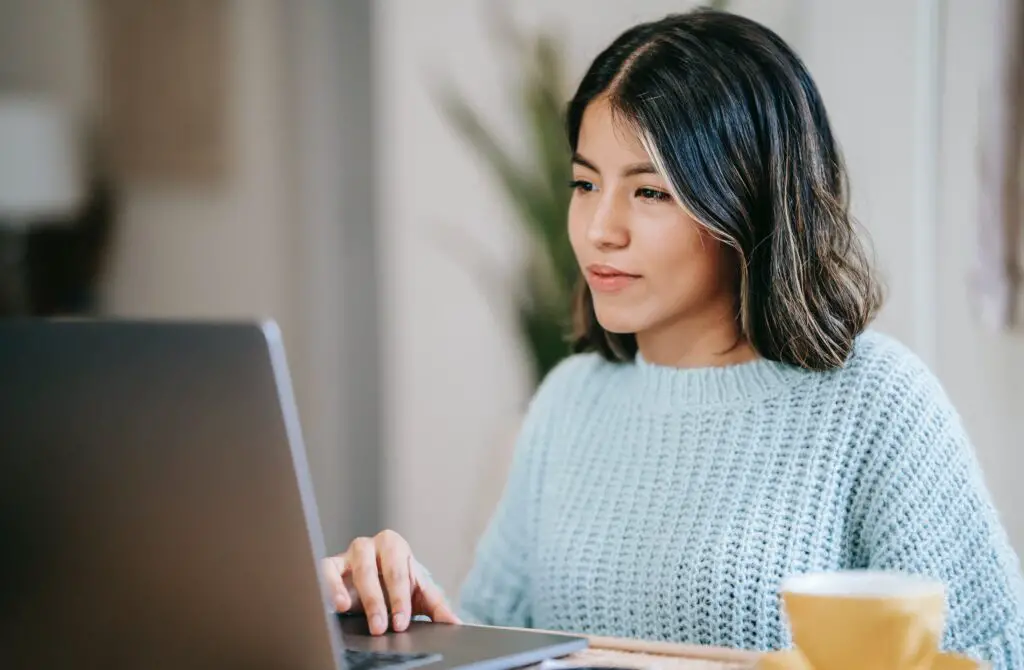
Assumptions for Chi-Square:
Amidst the intricacies of statistical analyses, it’s crucial to discern the bedrock assumptions upon which the edifice of Chi-square analysis is erected. Let’s illuminate these assumptions, illuminating the path for those navigating the realm of statistical inference:
- Independence of Observations:
At the heart of Chi-square analysis lies the assumption of independence among observations. Each data point should be autonomous, unaffected by the presence or absence of others. In essence, the outcome of one observation should not influence the outcome of another. This assumption ensures that the frequencies observed in different categories are not contingent upon each other, fostering the integrity of Chi-square analysis. - Random Sampling:
The second cornerstone of Chi-square analysis rests upon the premise of random sampling. For the results of Chi-square to be reliable and generalizable, the data should be derived from a random sample, ensuring that each member of the population has an equal chance of being selected. Random sampling mitigates the risk of bias, bolstering the validity of inferences drawn from Chi-square analysis. - Sufficient Sample Size:
Another crucial assumption underlying Chi-square analysis is the presence of a sufficiently large sample size. A small sample size can undermine the statistical power of Chi-square tests, rendering them prone to Type I or Type II errors. Adequate sample size not only enhances the precision of estimates but also upholds the assumptions of asymptotic theory, which underlie the Chi-square distribution. - Appropriate Measurement Scale:
Chi-square analysis necessitates categorical data, where observations are classified into discrete categories or groups. It is imperative that the variables under consideration are measured on a nominal or ordinal scale, aligning with the requirements of Chi-square tests. Continuous variables must be appropriately categorized to facilitate their utilization in Chi-square analysis, ensuring compatibility with its assumptions. - Expected Cell Frequencies:
A fundamental assumption of Chi-square analysis pertains to the presence of expected cell frequencies greater than or equal to five. When conducting Chi-square tests, each cell in the contingency table should ideally have an expected frequency of at least five to uphold the validity of the test statistics. This assumption ensures the reliability of Chi-square tests, averting distortions caused by sparse data. - Absence of Outliers:
Chi-square analysis operates under the assumption of homogeneity, presupposing uniformity within categories. Outliers or extreme values within the data can disrupt this homogeneity, potentially skewing the results of Chi-square tests. Therefore, it is imperative to screen for outliers and address them appropriately to safeguard the integrity of Chi-square analysis.
In essence, the efficacy of Chi-square analysis hinges upon the adherence to these fundamental assumptions, each serving as a linchpin in the process of statistical inference. By recognizing and upholding these assumptions, researchers can harness the full potential of Chi-square analysis, illuminating the intricacies of categorical data and unraveling the underlying patterns with precision and rigor.