Unveiling the Marvels of Machine Learning:
A Modern Perspective
In the cacophony of technological advancements that shape our world, one term stands out like a beacon of innovation: Machine Learning. It’s not merely a buzzword anymore; it’s the powerhouse driving everything from virtual assistants to self-driving cars. But what exactly is machine learning, and why does it hold such sway over our digital landscape? Let’s embark on a journey to unravel its mysteries.
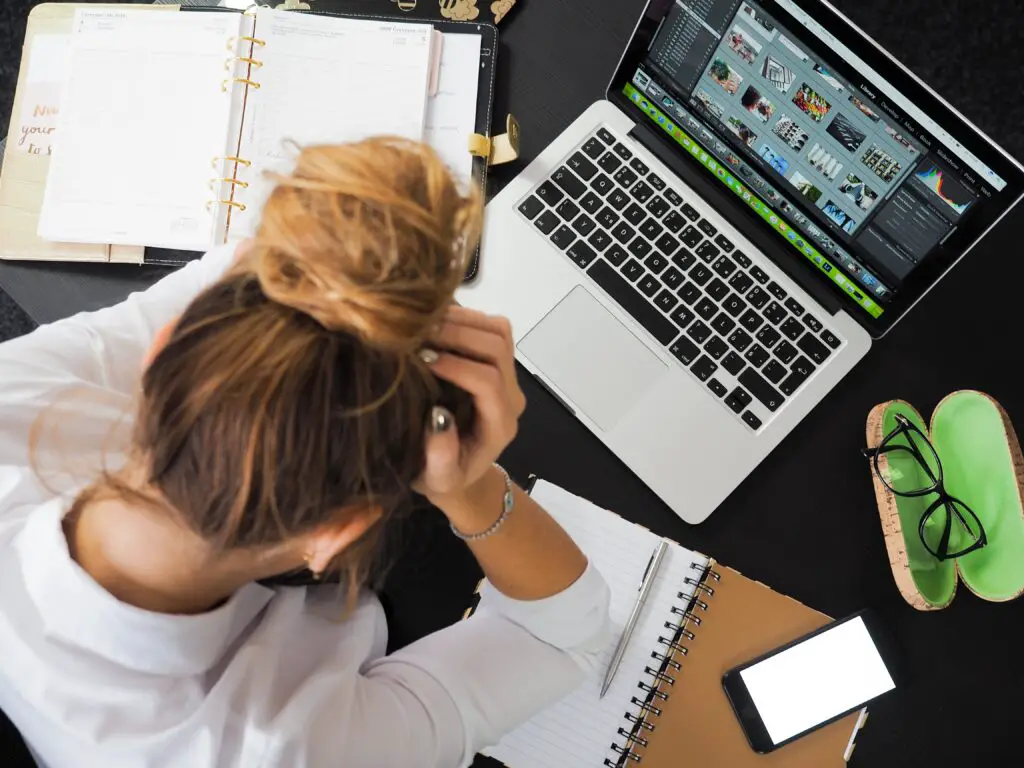
Definition for Machine Learning
At its core, machine learning is the art and science of empowering machines to learn from data without being explicitly programmed. Unlike traditional algorithms that follow rigid instructions, machine learning algorithms have the uncanny ability to adapt and improve their performance over time, much like how humans learn from experience.
Unpacking the Essence
Machine learning operates on the premise of extracting patterns and insights from vast amounts of data. Imagine feeding a computer system with heaps of labeled images—cats, dogs, and everything in between. Through exposure to this data, the machine learns to distinguish between different objects, gradually refining its ability to classify new images.
The Three Pillars: Supervised, Unsupervised, and Reinforcement Learning
Machine learning methodologies primarily fall into three categories: supervised learning, unsupervised learning, and reinforcement learning.
- Supervised Learning: This approach involves training a model on a labeled dataset, where each input is paired with the corresponding correct output. The algorithm learns to map inputs to outputs, enabling it to make predictions on unseen data accurately.
- Unsupervised Learning: Here, the algorithm delves into unlabeled data to uncover hidden patterns or structures. Without explicit guidance, the system identifies similarities or anomalies, clustering data points into meaningful groups or reducing the dimensionality of the dataset.
- Reinforcement Learning: Inspired by behavioral psychology, reinforcement learning revolves around the concept of rewards and punishments. The algorithm learns by interacting with an environment, receiving feedback in the form of rewards for desirable actions and penalties for unfavorable ones. Through trial and error, the system hones its decision-making prowess to maximize cumulative rewards.
The Tools of the Trade
Machine learning algorithms come in various shapes and sizes, each tailored to address specific tasks and challenges. From classic models like linear regression and decision trees to sophisticated deep learning architectures such as convolutional neural networks and recurrent neural networks, the arsenal of machine learning tools continues to expand, fueled by relentless innovation.
The Data Deluge
Central to the success of any machine learning endeavor is the availability of high-quality data. Terabytes of structured and unstructured data flood the digital landscape, encompassing everything from text and images to sensor readings and financial transactions. Harnessing this data treasure trove requires robust data collection, preprocessing, and cleaning pipelines to ensure that the input fed to machine learning models is pristine and devoid of biases.
Ethical Considerations
As machine learning permeates every facet of our lives, it brings forth a myriad of ethical considerations. From algorithmic bias and data privacy concerns to the socio-economic implications of automation, the ethical dimensions of machine learning demand careful deliberation and proactive measures to mitigate potential risks.
The Evolution Continues
Machine learning is not a static field but rather a dynamic ecosystem characterized by continuous evolution. As researchers push the boundaries of what’s possible, breakthroughs in areas like transfer learning, federated learning, and explainable AI pave the way for a future where machines not only learn but also understand and reason.
Conclusion
Machine learning, with its blend of artistry and scientific rigor, stands as a testament to humanity’s quest for knowledge and innovation. In its embrace, we find the promise of smarter algorithms, personalized experiences, and groundbreaking discoveries. As we navigate the ever-expanding landscape of machine learning, let us tread carefully, guided by the twin beacons of progress and ethical stewardship.