Unraveling the Intricacies of Box Plot Skewness
In the ever-evolving realm of data analysis, box plots stand as stalwart visual aids, offering profound insights into the distribution and dispersion of data points. Yet, within the seemingly simplistic confines of these rectangular representations lie nuances waiting to be deciphered. Among these subtleties, one often encounters the concept of box plot skewness, a metric that unveils the asymmetrical tendencies lurking within datasets. Let us embark on a journey to unravel the intricacies of box plot skewness, delving into its significance, calculation, and interpretation.
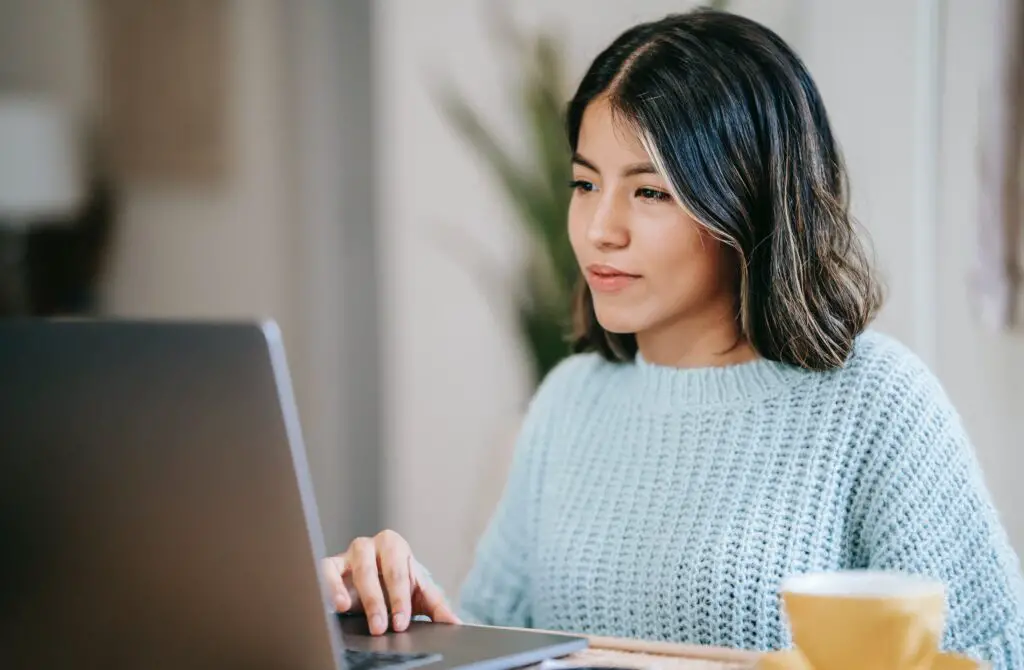
Box Plot Skewness:
Shedding Light on Asymmetry
At its core, box plot skewness serves as a beacon illuminating the asymmetrical nature of data distributions. While the traditional box plot provides a visual snapshot of the median, quartiles, and outliers, skewness adds another layer of understanding by indicating the extent to which the data deviates from symmetry. In essence, it offers a quantitative measure of the imbalance between the tails of the distribution, elucidating whether the bulk of the data clusters towards one end or is evenly spread.
Understanding Box Plot Skewness:
A Direct Answer
In its essence, box plot skewness provides a direct answer to a crucial question: How skewed is the distribution represented by the box plot? This metric quantifies the asymmetry of the distribution, offering insights into the shape and spread of the data. The calculation of box plot skewness involves examining the relative lengths of the whiskers, position of the median, and the distribution of data points within the quartiles. By evaluating these components, one can determine the degree of skewness and infer the underlying characteristics of the dataset.
Calculating Box Plot Skewness:
Unveiling the Methodology
To calculate box plot skewness, one typically employs statistical techniques such as moment-based formulas or computational algorithms. These methods involve assessing the moments of the distribution, particularly the third moment, which encapsulates skewness. By analyzing the position and dispersion of data points within the box plot, alongside the magnitude of outliers, statisticians derive a numerical value indicative of skewness. This value serves as a quantitative measure, allowing analysts to gauge the extent of asymmetry within the dataset accurately.
Interpreting Box Plot Skewness:
Deciphering the Insights
Upon obtaining the skewness coefficient, the task of interpretation unfolds, offering valuable insights into the nature of the dataset. A skewness value of zero signifies perfect symmetry, indicating a distribution where the tails extend equally in both directions from the median. Positive skewness implies that the right tail of the distribution is longer, indicating a prevalence of higher values, while negative skewness suggests a longer left tail and a preponderance of lower values. By discerning the direction and magnitude of skewness, analysts gain a deeper understanding of the underlying data dynamics.
Implications of Box Plot Skewness:
Navigating Analytical Terrain
In the realm of data analysis and interpretation, box plot skewness holds profound implications for decision-making and inference. Understanding the asymmetrical tendencies within a dataset enables analysts to tailor their analytical approaches accordingly. For instance, in finance, recognizing positive skewness in stock returns might inform risk management strategies, whereas negative skewness could influence portfolio optimization techniques. Similarly, in healthcare, identifying skewed distributions of patient outcomes can guide treatment protocols and resource allocation efforts. By embracing the insights garnered from box plot skewness, practitioners navigate the analytical terrain with heightened acuity and efficacy.
Unveiling Hidden Patterns:
Harnessing the Power of Skewness
Beyond its immediate applications in data analysis, box plot skewness unveils hidden patterns and anomalies that might evade superficial scrutiny. By peering beyond the confines of central tendency and dispersion, analysts unearth the idiosyncrasies embedded within datasets, paving the way for informed decision-making and predictive modeling. Whether unraveling the intricacies of financial markets, deciphering consumer behavior, or unraveling the mysteries of natural phenomena, the discernment of skewness serves as a guiding light, illuminating the path towards deeper insights and actionable intelligence.
Conclusion
In the realm of data analysis, the exploration of box plot skewness transcends mere statistical calculation, embodying a quest for understanding amidst complexity. By scrutinizing the asymmetrical tendencies within datasets, analysts unearth a wealth of insights that inform decision-making, drive innovation, and unravel the mysteries of the world around us. As we continue to navigate the intricate landscape of data analytics, let us embrace the power of skewness, harnessing its transformative potential to illuminate the path towards knowledge and enlightenment.