In the Realm of Statistical Tests:
Unveiling the T Test vs. Mann-Whitney U Test
In the vast landscape of statistical analysis, where data reigns supreme and conclusions are drawn with meticulous scrutiny, two prominent figures stand tall: the T test and the Mann-Whitney U test. These statistical methods serve as formidable tools in the arsenal of researchers and analysts, enabling them to unravel the mysteries hidden within data sets and draw meaningful insights. But amidst their shared goal of testing hypotheses and uncovering truths, lies a distinct contrast in their approaches and applications. Join me on a journey through the realm of statistical tests as we delve into the nuanced differences between the T test and the Mann-Whitney U test.
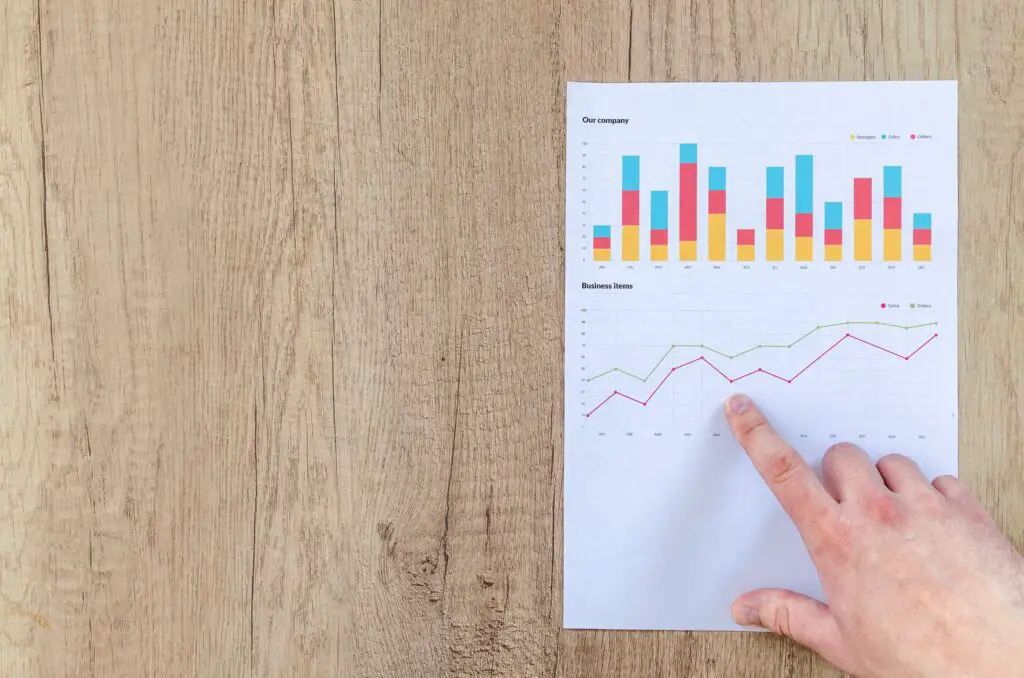
Unveiling the T Test vs. Mann-Whitney U Test
Let’s begin our exploration by shedding light on the fundamental question at hand: What sets the T test apart from the Mann-Whitney U test? At their core, both tests are designed to assess the significance of differences between groups or samples. However, their methodologies diverge, paving the way for distinct scenarios where each test shines.
The T test, a stalwart in the realm of inferential statistics, is revered for its efficacy in comparing the means of two independent groups. Whether you’re investigating the impact of a new drug on patient outcomes or examining the efficacy of different teaching methods on student performance, the T test emerges as a reliable ally. It operates under the assumption of normally distributed data, leveraging the t-distribution to evaluate whether the observed differences in sample means are statistically significant or simply due to chance.
Conversely, the Mann-Whitney U test takes a different route, offering solace when the assumptions of normality are violated or when dealing with ordinal or non-normally distributed data. As a non-parametric test, it eschews reliance on specific distributional assumptions, making it robust in scenarios where the T test may falter. Instead of scrutinizing means, the Mann-Whitney U test focuses on medians, assessing whether two independent samples are drawn from populations with the same distribution. Its resilience in the face of non-normality renders it a versatile tool across diverse fields, from social sciences to ecology, where data often defy the constraints of normality.
Deciphering the Dichotomy:
When to Choose T or U?
Now that we’ve unraveled the essence of both tests, the pressing question arises: When should you opt for the T test, and when does the Mann-Whitney U test steal the spotlight?
T Test:
- Embrace the T test when you’re dealing with continuous data and seeking to compare means between two independent groups.
- Rely on its robustness when the assumptions of normality are met, ensuring accurate inference regarding population parameters.
- Harness its power in scenarios where the sample size is sufficient to uphold the central limit theorem, bolstering the validity of results.
Mann-Whitney U Test:
- Turn to the Mann-Whitney U test when confronted with non-normally distributed data or when the sample size is small.
- Leverage its non-parametric nature to sidestep the shackles of distributional assumptions, ensuring reliable inference regardless of data distribution.
- Embrace its versatility in analyzing ordinal or ranked data, transcending the confines of traditional parametric tests.
Beyond the Basics: Caveats and Considerations
While the T test and the Mann-Whitney U test stand as pillars of statistical inference, navigating the terrain of hypothesis testing demands a nuanced understanding of their limitations and caveats.
T Test:
- Exercise caution when the assumptions of normality are violated, as this can undermine the validity of results and lead to erroneous conclusions.
- Verify the equality of variances between groups, especially in the context of independent samples, to uphold the assumptions of homogeneity of variances.
Mann-Whitney U Test:
- Acknowledge the loss of efficiency compared to the T test, particularly when dealing with normally distributed data and larger sample sizes.
- Interpret results with caution in scenarios with tied ranks or extreme outliers, as these can influence the accuracy of the test.
Conclusion
In the ever-evolving landscape of statistical analysis, the choice between the T test and the Mann-Whitney U test hinges on a myriad of factors, from data distribution to sample size and the nature of the research question at hand. While the T test commands authority in the realm of normally distributed data and ample sample sizes, the Mann-Whitney U test emerges as a stalwart in the face of non-normality and smaller sample sizes. Armed with this understanding, researchers and analysts can navigate the complexities of hypothesis testing with confidence, wielding the appropriate test to unveil the truths hidden within their data.