T Test vs Chi-Square Test:
Unraveling the Statistical Conundrum
In the labyrinth of statistical analysis, two stalwarts stand tall, each wielding its own set of principles and applications: the venerable t-test and the formidable chi-square test. These statistical tools serve as guiding stars for researchers and analysts navigating the vast sea of data, offering clarity amidst the complexities. But what sets them apart? How do they diverge in their methodologies, and when should one be favored over the other? Let us embark on a journey of discovery, delving into the nuances of the t-test and the chi-square test, unraveling their mysteries to illuminate the path towards informed decision-making.
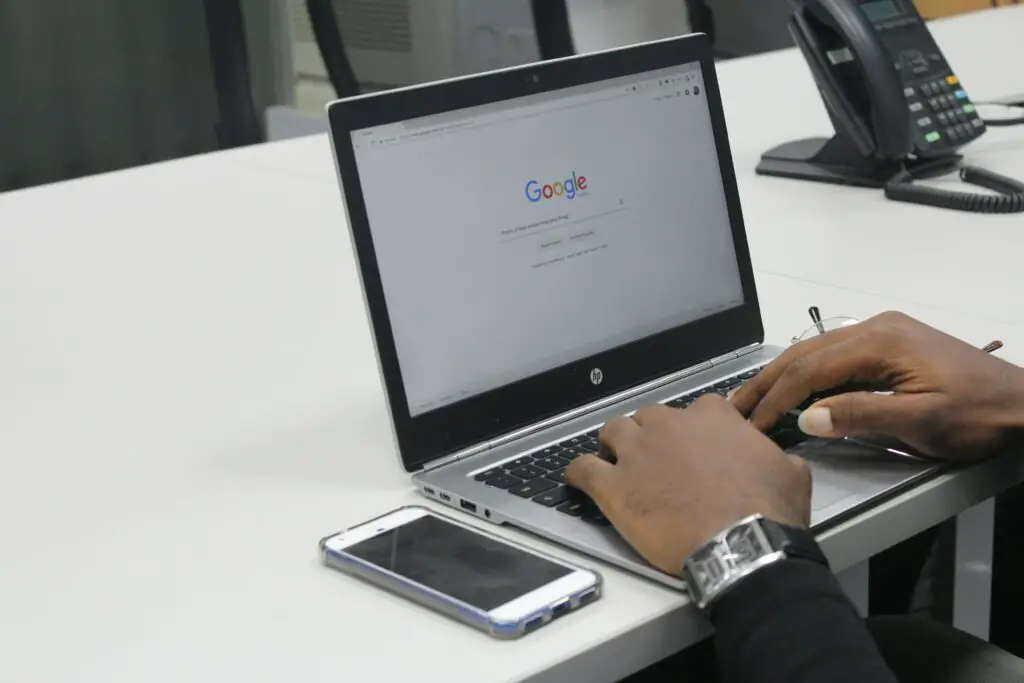
Paving the Way
In the realm of statistics, uncertainty reigns supreme. Researchers and analysts grapple with data, seeking patterns, trends, and relationships that provide insight into the phenomena under study. Amidst this quest for understanding, statistical tests emerge as indispensable tools, offering a systematic framework for drawing conclusions from empirical evidence. Among these tools, the t-test and the chi-square test occupy prominent positions, each serving distinct purposes in the arsenal of statistical analysis.
T Test vs Chi-Square Test:
Deciphering the Dichotomy
The T Test: A Measure of Means
At the heart of the t-test lies the concept of means – a numerical summary that encapsulates the central tendency of a dataset. This venerable test, devised by the illustrious William Sealy Gosset under the pseudonym “Student,” serves as a stalwart companion in the realm of hypothesis testing, particularly when dealing with small sample sizes. Whether comparing the means of two independent groups or assessing the significance of a single sample mean against a known value, the t-test emerges as a steadfast ally, providing a robust framework for inference.
The Chi-Square Test: Champion of Categorical Data
Contrastingly, the chi-square test operates in a realm governed by categorical variables, where frequencies and proportions reign supreme. Originating from the fertile mind of Karl Pearson, this test epitomizes the marriage between observed and expected frequencies, scrutinizing their alignment to discern patterns and dependencies within the data. Whether unraveling the mysteries of association in contingency tables or probing the goodness-of-fit of a theoretical model to empirical data, the chi-square test stands as a beacon of statistical rigor.
T Test vs Chi-Square Test:
Head-to-Head
Comparing Means vs. Assessing Independence
At the crux of the dichotomy between the t-test and the chi-square test lies the distinction between continuous and categorical data. The t-test, with its focus on means and numerical variables, finds its niche in scenarios where researchers seek to discern differences between groups or ascertain the significance of a sample mean. On the other hand, the chi-square test thrives in environments where categorical variables hold sway, facilitating the exploration of relationships, associations, and proportions within the data.
Small Samples vs. Categorical Counts
Another pivotal consideration in the t-test vs. chi-square test dilemma pertains to sample size. While the t-test retains its efficacy even in the presence of small sample sizes, the chi-square test demonstrates robustness in scenarios where categorical counts abound. Thus, researchers must exercise prudence in selecting the appropriate test based on the nature of their data and the underlying research question.
Parametric Assumptions vs. Distribution-Free Testing
Furthermore, the t-test operates under the umbrella of parametric assumptions, necessitating adherence to conditions such as normality and homogeneity of variance. In contrast, the chi-square test offers a refuge for those navigating the murky waters of non-normal distributions, embracing the mantra of distribution-free testing. This flexibility renders the chi-square test a versatile tool in scenarios where parametric assumptions falter, offering solace to researchers grappling with real-world data.
Navigating the Statistical Landscape
In the realm of statistical analysis, the choice between the t-test and the chi-square test transcends mere methodological preferences; it embodies a strategic decision with far-reaching implications. As researchers traverse the statistical landscape, armed with data and hypotheses, they must tread cautiously, mindful of the intricacies that underpin each test. Whether illuminating the nuances of means or unraveling the mysteries of categorical data, the t-test and the chi-square test stand as pillars of statistical inference, guiding the way towards enlightenment amidst the fog of uncertainty.
Conclusion
In the tapestry of statistical analysis, the t-test and the chi-square test emerge as threads of distinction, weaving together a narrative of insight and discovery. Through their distinct methodologies and applications, these tests offer researchers a roadmap for navigating the complexities of empirical inquiry. As the journey unfolds, it is not merely a question of choosing between two statistical tools; rather, it is a quest for understanding, a pursuit of knowledge amidst the ebb and flow of data. So, let us embrace the dichotomy, embrace the challenge, and embark on a voyage of statistical enlightenment.