Understanding Statistics and Parameters:
Unveiling the Essence of Data
In the vast realm of data analysis and statistical inference, two fundamental concepts reign supreme: statistics and parameters. They form the backbone of quantitative research, guiding us through the labyrinth of numbers to uncover meaningful insights about the world around us. Yet, despite their ubiquity, the distinction between statistics and parameters can often be shrouded in ambiguity. In this elucidating journey, we embark on a quest to unravel the essence of statistics and parameters, shedding light on their roles, differences, and significance in the realm of data analysis.
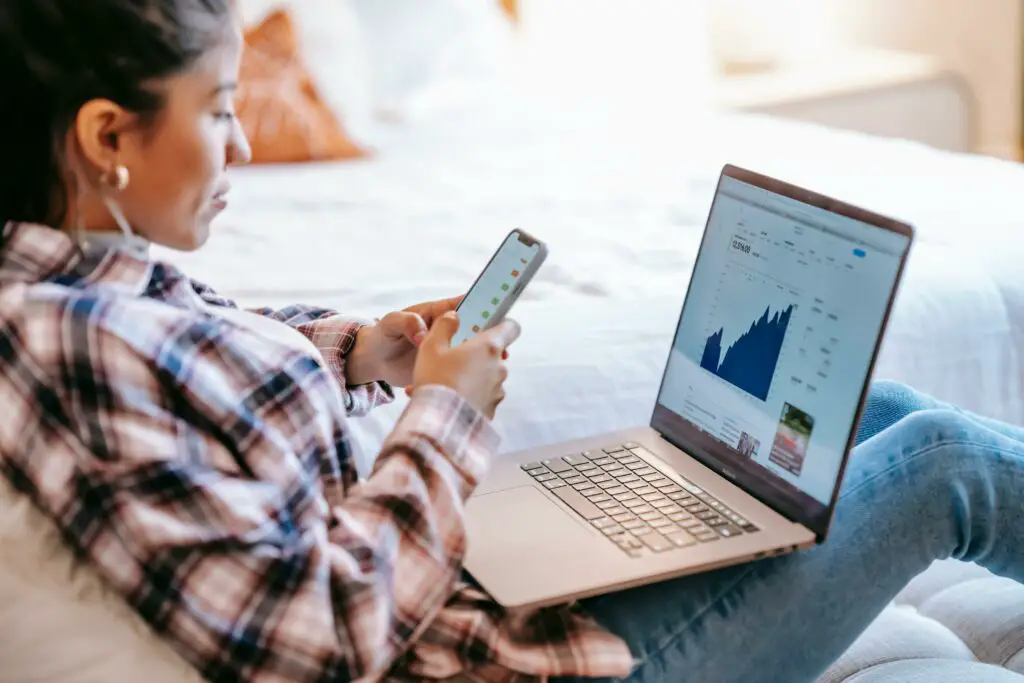
Statistical vs. Parameter:
Demystifying the Duality
At the heart of statistical analysis lies the dichotomy between statistics and parameters. To understand this duality, let us delve into their definitions and roles in the realm of data analysis.
Statistics:
The Quest for Understanding
Statistics, in its essence, embodies the art of summarizing and interpreting data. When we speak of statistics, we refer to numerical values calculated from a sample—a subset of the population under study. These values serve as estimators, offering insights into the characteristics of the larger population from which the sample is drawn.
Imagine you’re conducting a survey to determine the average income of households in a city. You randomly select 500 households and calculate the average income based on their responses. This resulting average income figure is a statistic. It represents an estimate of the true average income of all households in the city, based on the information gleaned from the sample.
Parameters:
The Pillars of Population
On the other hand, parameters represent the true, fixed characteristics of a population. Unlike statistics, which are derived from samples, parameters encapsulate the intrinsic properties of the entire population under study. They serve as immutable constants, defining the population’s attributes with unwavering precision.
Continuing with our example, suppose you want to determine the exact average income of all households in the city, not just those surveyed. This unchanging value, reflecting the population’s true average income, is the parameter. It stands as the gold standard against which sample statistics are compared, providing a benchmark for evaluating the reliability and accuracy of our estimates.
The Fundamental Distinction:
Sample vs. Population
At the heart of the statistical vs. parameter debate lies the distinction between sample and population. A sample represents a subset of the population—a smaller, manageable slice chosen to represent the whole. In contrast, the population encompasses the entirety of individuals or elements under study, embodying the full spectrum of variability and diversity inherent in the phenomenon of interest.
Statistics arise from the analysis of samples, offering glimpses into the population’s characteristics through inference and estimation. Parameters, on the other hand, emanate from the population itself, embodying its intrinsic properties with unwavering fidelity. While statistics fluctuate from sample to sample, parameters stand as immutable constants, defining the essence of the population with unyielding precision.
Navigating Uncertainty:
Precision vs. Accuracy
In the realm of data analysis, precision and accuracy serve as guiding beacons, illuminating the path towards reliable inference and meaningful insights. Understanding the interplay between statistics and parameters is crucial for navigating the labyrinth of uncertainty and ensuring the validity of our conclusions.
Precision refers to the consistency and reproducibility of our measurements or estimates. In the context of statistics, precision relates to the variability of sample estimates around the true parameter value. A statistic is considered precise if repeated sampling yields similar results, clustering closely around the population parameter.
Accuracy, on the other hand, pertains to the closeness of our estimates to the true, population parameter. While precision speaks to the consistency of our estimates, accuracy delves into their correctness, gauging how closely they align with the population’s true characteristics.
Striking a Delicate Balance:
Bias and Variability
In the pursuit of meaningful inference, researchers must grapple with the twin specters of bias and variability, striving to strike a delicate balance between accuracy and precision. Bias, stemming from systematic errors or distortions in the sampling process, tilts our estimates away from the true population parameter, leading to erroneous conclusions.
Variability, on the other hand, embodies the inherent fluctuations and randomness observed in sample statistics. While variability is an inherent feature of sampling, excessive variability can undermine the reliability of our estimates, casting doubt on the validity of our conclusions.