Unraveling the Enigma of Spearman Correlation
In the intricate labyrinth of statistics, where numbers dance in harmony or discord, lies the Spearman correlation—a beacon guiding researchers through the murky waters of data analysis. In this article, we embark on a journey to unravel the mysteries surrounding this statistical measure, understanding its significance, applications, and implications in modern research and beyond.
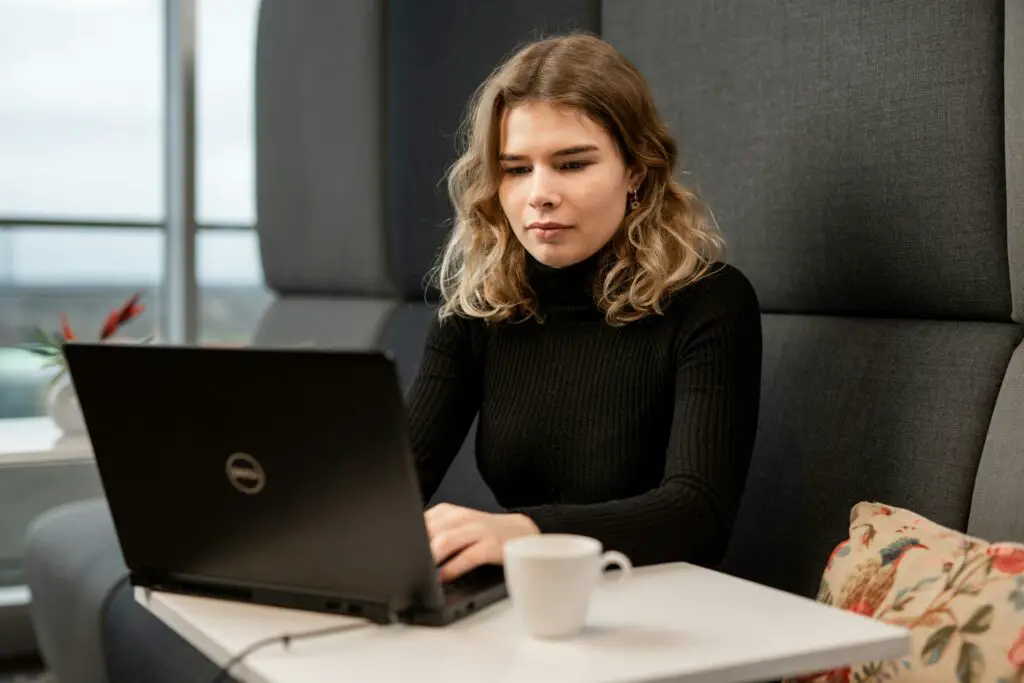
Spearman Correlation:
Spearman Correlation Unveiled
At its core, the Spearman correlation is a statistical method used to measure the strength and direction of association between two ranked variables. Named after Charles Spearman, the British psychologist who pioneered its development, this correlation coefficient transcends the confines of ordinary Pearson correlation by accommodating non-linear relationships and variables measured on ordinal scales.
Deciphering the Coefficient
Unlike its Pearson counterpart, which operates on interval or ratio scale data, Spearman correlation operates on rank-ordered data. This means that instead of relying on exact numerical values, it examines the relative positions of data points within each variable. The resulting coefficient, denoted by ρ (rho), ranges from -1 to 1, with -1 signifying a perfect negative monotonic relationship, 1 indicating a perfect positive monotonic relationship, and 0 suggesting no monotonic relationship between the variables.
Applications in Research and Beyond
The utility of Spearman correlation extends across various domains, from psychology and sociology to economics and environmental science. In psychology, researchers employ it to analyze the relationship between ordinal survey responses, such as Likert scale ratings, shedding light on patterns of human behavior and cognition. Similarly, in economics, Spearman correlation aids in identifying trends and dependencies among ranked economic indicators, guiding policymakers in making informed decisions.
Limitations and Considerations
However, like any statistical tool, Spearman correlation comes with its caveats. It assumes a monotonic relationship between variables, which might not always hold true in real-world scenarios. Moreover, its sensitivity to outliers and sample size warrants cautious interpretation, urging researchers to complement its findings with additional analyses for robust conclusions.
Navigating the Statistical Seas
In the vast ocean of statistical methods, Spearman correlation serves as a steadfast compass, guiding researchers through turbulent waves of data. Its versatility, simplicity, and resilience against the constraints of traditional correlation coefficients make it an indispensable tool in the arsenal of every data scientist, analyst, and researcher.
Conclusion
As we navigate the intricate landscape of statistics, Spearman correlation emerges as a beacon of clarity, illuminating the path towards deeper insights and understanding. From unraveling the complexities of human behavior to deciphering economic trends, its applicability knows no bounds, transcending disciplines and enriching our collective pursuit of knowledge. So, let us embrace the elegance of Spearman correlation, forging ahead in our quest to decipher the enigmatic language of data.