Unraveling the Null Hypothesis in Chi-Square Analysis
In the vast landscape of statistical analysis, where numbers dance and patterns emerge, the chi-square test stands as a stalwart, helping researchers discern the significance of observed data. At its heart lies a critical concept: the null hypothesis. Let us embark on a journey to demystify this cornerstone of chi-square analysis, exploring its essence, implications, and applications.
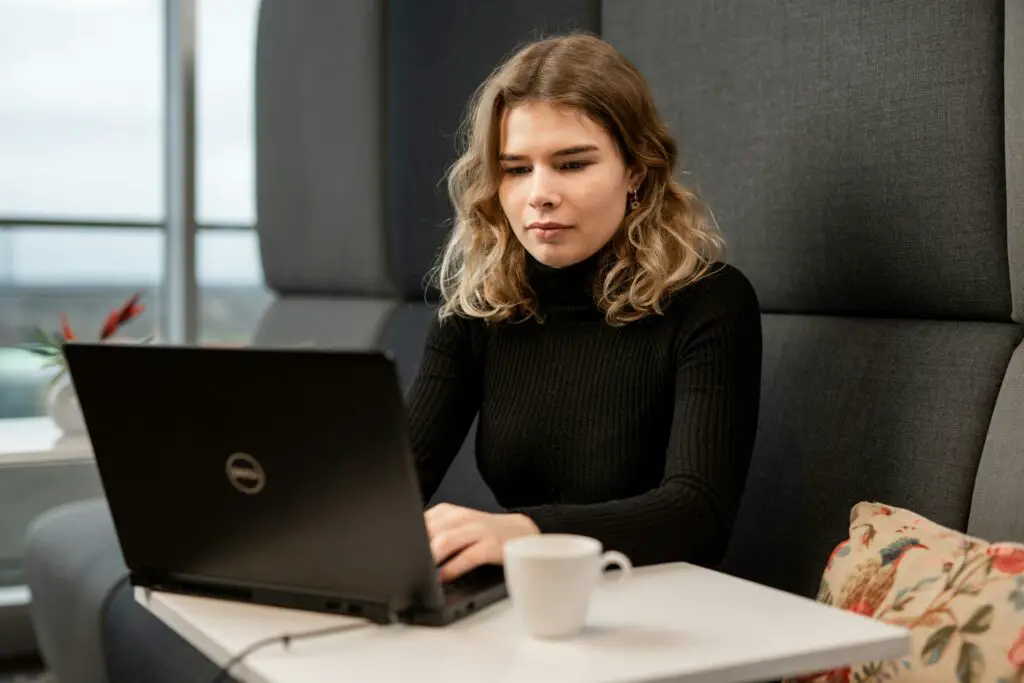
Null Hypothesis in Chi-Square:
Unveiling the Essence
At its core, the null hypothesis in chi-square analysis posits that there is no significant difference between the observed and expected frequencies of a categorical variable. In simpler terms, it suggests that any deviation between what we expect to observe and what we actually observe is due to chance alone, rather than any true effect or relationship.
This hypothesis serves as the null point against which researchers gauge the validity of their findings. It embodies skepticism, challenging researchers to substantiate any claims of association or difference in frequencies within their data. By subjecting their hypotheses to rigorous scrutiny, researchers ensure that their conclusions are grounded in empirical evidence rather than mere conjecture.
Understanding Chi-Square Analysis
Before delving deeper into the null hypothesis, let’s acquaint ourselves with the chi-square test itself. Named for the Greek letter “χ²” (chi-square), this statistical method evaluates the distribution of categorical data and assesses whether any observed differences are statistically significant.
In essence, chi-square analysis compares observed frequencies in different categories to the frequencies we would expect to see if there were no association between the variables being studied. It quantifies the extent of deviation from expected frequencies, providing researchers with a measure of the likelihood that such deviation occurred purely by chance.
Embracing the Null Hypothesis:
A Guiding Principle
Within the realm of chi-square analysis, the null hypothesis serves as both a guiding principle and a formidable adversary. Its assertion of no significant difference challenges researchers to scrutinize their data rigorously, employing statistical tools to discern genuine patterns from random fluctuations.
When conducting a chi-square test, researchers formulate two hypotheses: the null hypothesis (H₀) and the alternative hypothesis (H₁). The null hypothesis posits no relationship or difference between variables, while the alternative hypothesis suggests the presence of such a relationship or difference.
In the context of chi-square analysis, the null hypothesis typically takes the form: “There is no significant difference between the observed and expected frequencies of the categorical variable.” Conversely, the alternative hypothesis might propose that a relationship exists, such as: “There is a significant difference between the observed and expected frequencies of the categorical variable.”
Interpreting Chi-Square Results:
The Dance of P-Values
Once the chi-square test is conducted, researchers turn their attention to the p-value—a numerical measure that quantifies the strength of evidence against the null hypothesis. A low p-value suggests that the observed deviation from expected frequencies is unlikely to occur purely by chance, leading researchers to reject the null hypothesis in favor of the alternative.
Conversely, a high p-value indicates that the observed deviation could plausibly occur by chance alone, failing to provide sufficient evidence to reject the null hypothesis. In such cases, researchers refrain from making definitive claims of association or difference, acknowledging the possibility that their findings may be due to random variation.
Applications and Extensions:
Beyond the Basics
While the null hypothesis in chi-square analysis finds widespread application in fields ranging from biology to social sciences, its significance extends beyond the confines of traditional statistical testing. Researchers often employ extensions of the chi-square test to analyze complex data sets, adapting its principles to suit the unique demands of their research questions.
From contingency table analysis to goodness-of-fit tests, the null hypothesis remains a steadfast companion, guiding researchers through the intricacies of categorical data analysis. Its resilience in the face of uncertainty underscores the importance of skepticism and empirical rigor in the pursuit of scientific knowledge.
Conclusion
In the realm of chi-square analysis, the null hypothesis serves as a beacon of skepticism, challenging researchers to scrutinize their findings with precision and diligence. By subjecting their hypotheses to rigorous testing, researchers ensure that their conclusions are anchored in empirical evidence rather than mere speculation.
As we navigate the intricate landscape of statistical analysis, let us heed the call of the null hypothesis, embracing its skepticism as a cornerstone of scientific inquiry. In doing so, we honor the pursuit of truth and uphold the integrity of empirical research for generations to come.