Understanding the Beauty of the Normal Distribution
In the vast symphony of statistics, there exists a melody so harmonious, so ubiquitous, that it orchestrates the rhythms of our everyday lives without us even realizing. This symphony is none other than the enchanting Normal Distribution, a concept so elegantly simple yet profoundly complex that it weaves its way through fields as diverse as economics, physics, psychology, and beyond. Let us embark on a journey to unravel the secrets of this captivating statistical concept, exploring its essence, its applications, and its enduring relevance in the tapestry of human knowledge.
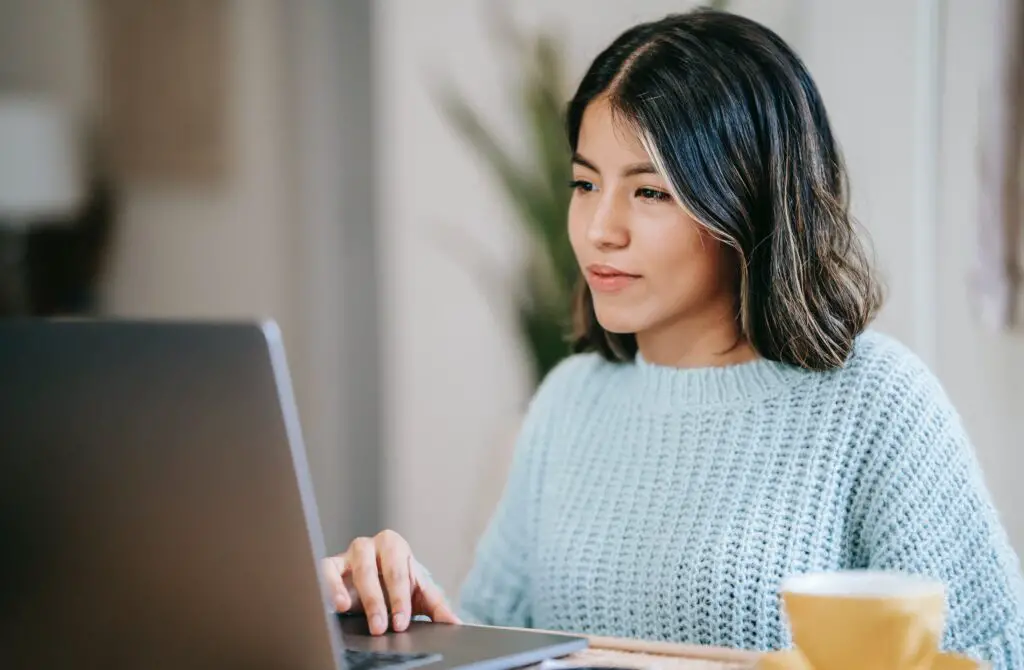
Normal Distribution:
A Primer
At the heart of the statistical realm lies the concept of the Normal Distribution, also known as the Gaussian distribution, after the brilliant mind of Carl Friedrich Gauss who first introduced it in the early 19th century. Picture a graceful curve, symmetrically rising and falling, with its peak at the center and its tails tapering off into infinity. This is the essence of the Normal Distribution, a bell-shaped curve that encapsulates the essence of randomness and variability in a multitude of phenomena.
Normal Distribution:
What is it?
The Normal Distribution, in its essence, is a probability distribution that is characterized by its bell-shaped curve. At its core, it represents the probability of various outcomes occurring, with the highest probability concentrated around the mean, or average, of the distribution. This means that in a perfectly normal distribution, most data points cluster around the mean, with progressively fewer occurring further away from it. The beauty of this distribution lies in its simplicity and its ability to model a wide array of natural phenomena, making it one of the most fundamental concepts in statistics.
Properties of the Normal Distribution
One of the defining characteristics of the Normal Distribution is its symmetry. The curve is perfectly symmetrical around its mean, meaning that the probabilities of events occurring to the left and right of the mean are equal. This symmetry lends the distribution its elegant shape and makes it an ideal candidate for modeling various real-world phenomena.
Another key property of the Normal Distribution is its parameterization by two parameters: the mean (μ) and the standard deviation (σ). The mean represents the central tendency of the distribution, while the standard deviation measures the spread or variability of the data around the mean. Together, these parameters allow us to fully describe the characteristics of the distribution and make predictions about the likelihood of different outcomes.
Applications of the Normal Distribution
The versatility of the Normal Distribution knows no bounds, as it finds applications in fields ranging from finance to biology, from engineering to social sciences. In finance, for example, the Normal Distribution is often used to model stock prices and returns, enabling investors to make informed decisions about risk and return. In biology, it can describe the distribution of traits within a population, shedding light on evolutionary processes and genetic variation.
In manufacturing processes, the Normal Distribution is used to ensure quality control by monitoring deviations from the mean and identifying potential defects. In psychology and education, it helps researchers understand phenomena such as intelligence and test scores, allowing for the development of standardized assessments and predictive models.
The Central Limit Theorem:
Unveiling the Magic
Central to the mystique of the Normal Distribution is the Central Limit Theorem, a concept so profound that it underpins much of modern statistical theory. In essence, the Central Limit Theorem states that the sum (or average) of a large number of independent and identically distributed random variables tends towards a Normal Distribution, regardless of the shape of the original distribution. This remarkable theorem is the reason why the Normal Distribution is ubiquitous in statistical inference, providing a robust framework for making inferences about populations based on sample data.
Normal Distribution in the Digital Age
In an era defined by data abundance and technological innovation, the Normal Distribution continues to reign supreme as a cornerstone of statistical analysis. With the advent of powerful computational tools and sophisticated algorithms, researchers and practitioners can harness the predictive power of the Normal Distribution to unlock insights and drive decision-making across a myriad of domains.
From predicting customer preferences in e-commerce to optimizing supply chain logistics, from modeling climate patterns to detecting anomalies in cybersecurity, the Normal Distribution remains an indispensable tool in the arsenal of modern data science. Its elegance and simplicity stand as a testament to the enduring power of mathematical abstraction in elucidating the complexities of the world around us.
Conclusion
In the grand tapestry of human knowledge, few concepts possess the enduring elegance and universal applicability of the Normal Distribution. From its humble origins in the musings of a 19th-century polymath to its pervasive presence in the digital age, the Normal Distribution continues to captivate and inspire generations of scholars, scientists, and practitioners alike. As we navigate the ever-changing landscape of data and discovery, let us never forget the beauty and brilliance of this timeless statistical concept, a beacon of certainty in a sea of uncertainty.