Unlocking the Mysteries of Statistical Analysis:
Kendall Tau-b vs. Spearman
In the realm of statistics, where numbers wield the power to reveal hidden truths and patterns, two prominent figures stand tall: Kendall Tau-b and Spearman. These statistical measures serve as the compass guiding researchers through the labyrinth of data, illuminating relationships and dependencies. But what sets them apart? How do they navigate the intricate landscape of correlations? Let’s embark on a journey to unravel the mysteries and nuances of Kendall Tau-b versus Spearman.
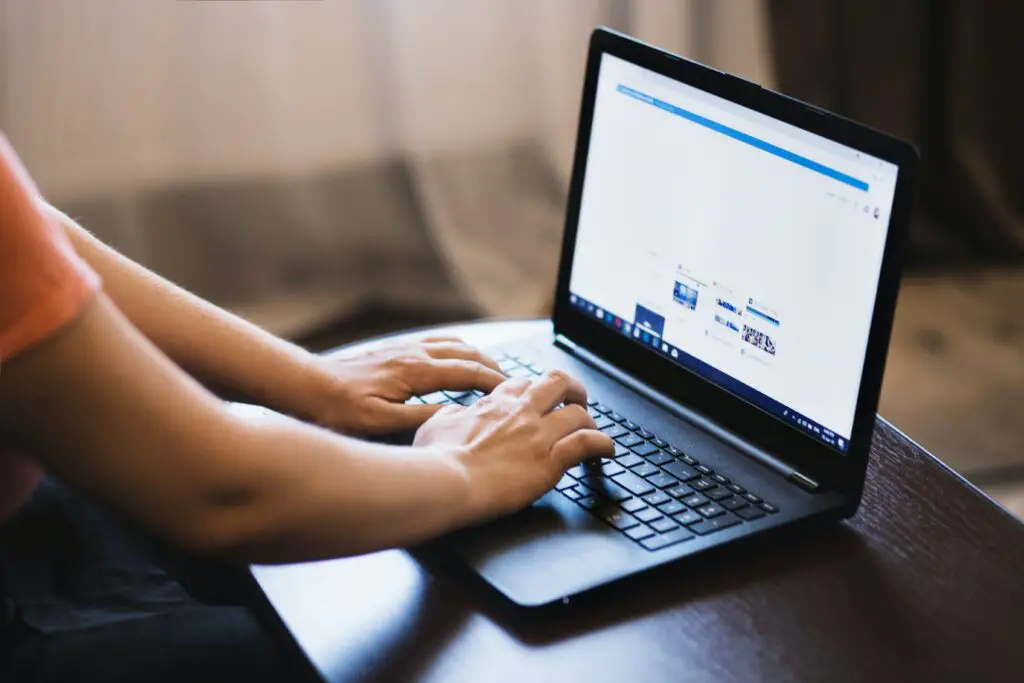
Navigating the Statistical Landscape
In the vast expanse of data analysis, understanding the dynamics between variables is paramount. Researchers and analysts seek to uncover the underlying connections that shape our world. Enter correlation analysis – the cornerstone of statistical exploration. At its core lies the quest to quantify the strength and direction of relationships between variables. Among the arsenal of correlation coefficients, Kendall Tau-b and Spearman emerge as stalwarts, each offering unique insights into the intricate dance of data points.
Kendall Tau-b vs. Spearman:
Decoding the Differences
Kendall Tau-b: Capturing the Essence of Concordance
Kendall Tau-b, a robust measure of correlation, strides onto the stage with an air of elegance and simplicity. Its prowess lies in assessing the degree of concordance between two variables, irrespective of the nature of their relationship. Imagine a scenario where we seek to determine the agreement between the rankings assigned to pairs of observations. Kendall Tau-b gracefully steps in, unfazed by the magnitude or scale of the data, to unveil the underlying harmony.
- Spearman: Spearheading the Charge*
In the arena of correlation analysis, Spearman stands as a venerable stalwart, wielding its spear to pierce through the veil of uncertainty. Renowned for its versatility, Spearman transcends the confines of linear relationships, offering a robust solution for assessing monotonic associations. Unlike Kendall Tau-b, Spearman evaluates the strength and direction of correlations based on the rank orders of observations. It navigates the terrain of non-parametric data with finesse, illuminating the path towards understanding the underlying dependencies.
Kendall Tau-b vs. Spearman:
A Direct Comparison
In the clash of statistical titans, how do Kendall Tau-b and Spearman fare? Let’s delve into a direct comparison to shed light on their distinctive features:
- Interpretation: Kendall Tau-b quantifies the degree of concordance between variables, emphasizing the proportion of concordant pairs relative to the total number of pairs. On the other hand, Spearman evaluates the monotonic relationship between variables, focusing on the direction and magnitude of rank-based associations.
- Sensitivity to Ties: Kendall Tau-b exhibits robustness in the face of tied observations, gracefully accommodating instances where multiple data points share identical ranks. In contrast, Spearman’s sensitivity to ties necessitates caution in scenarios characterized by tied ranks, as it may inflate the correlation coefficient.
- Scope of Application: While both Kendall Tau-b and Spearman offer valuable insights into correlations, their applicability varies depending on the nature of the data and research objectives. Kendall Tau-b excels in scenarios where the focus lies on assessing concordance, making it ideal for studies involving rankings or ordinal data. Spearman, with its emphasis on monotonic relationships, proves invaluable in analyzing variables with non-linear associations or skewed distributions.
In essence, the choice between Kendall Tau-b and Spearman hinges on the specific nuances of the research question and the underlying characteristics of the data. While Kendall Tau-b embodies the essence of concordance, Spearman spearheads the exploration of monotonic associations, each offering a unique perspective on the complex interplay of variables.
Exploring the Practical Implications
Beyond the realm of theoretical discourse, understanding the practical implications of choosing between Kendall Tau-b and Spearman is paramount. Consider a scenario where researchers seek to evaluate the correlation between the rankings assigned by two groups of experts in a subjective evaluation task. In this context, Kendall Tau-b emerges as the preferred choice, offering a robust measure of concordance that aligns with the research objectives.
Conversely, imagine a study investigating the relationship between the academic performance of students and their self-reported study hours. Here, Spearman takes center stage, adeptly capturing the monotonic trend between study hours and academic achievement, regardless of the specific rank assignments.
Conclusion
As we navigate the labyrinthine landscape of statistical analysis, Kendall Tau-b and Spearman stand as beacons of insight, guiding us through the intricate web of correlations. From the elegance of concordance to the depth of monotonic associations, each statistical measure offers a unique lens through which to decipher the complexities of data relationships. So, whether you find yourself immersed in the world of rankings or exploring the nuances of non-linear dependencies, rest assured that Kendall Tau-b and Spearman await, ready to illuminate the path forward.