Homoscedasticity:
Understanding Variability in Statistical Analysis
In the labyrinth of statistical analysis, where numbers dance and patterns emerge, lies a concept that whispers tales of variability—homoscedasticity. It’s a term that may sound like a mouthful, but its essence is essential for anyone diving into the depths of data analysis. In this article, we embark on a journey to unravel the mysteries of homoscedasticity, exploring its meaning, significance, and implications in statistical analysis.
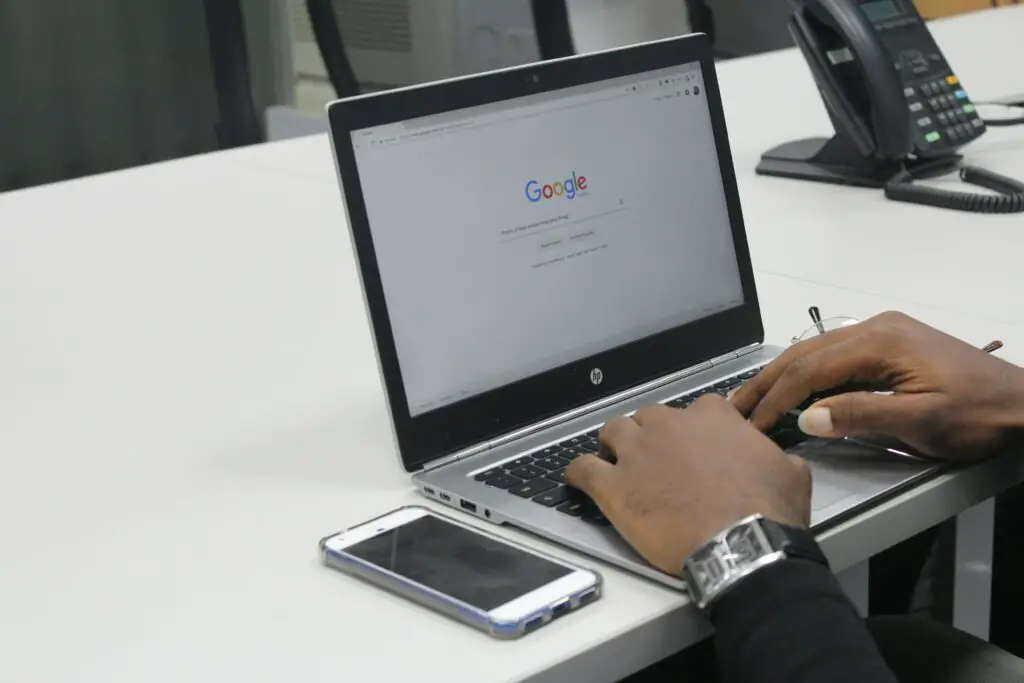
Homoscedasticity
Homoscedasticity: a term that might evoke images of a complex mathematical landscape, yet at its core lies a simple concept. Homoscedasticity, derived from the Greek words “homo,” meaning same, and “skedasis,” meaning dispersion, refers to a fundamental assumption in statistics. It entails the equality of variances across different levels of an independent variable.
What is Homoscedasticity?
In the realm of statistics, homoscedasticity is the assumption that the variability, or spread, of the dependent variable is consistent across all levels of the independent variable(s). Picture a scatter plot where the points are scattered evenly around a regression line, exhibiting a uniform dispersion of data points. This is the essence of homoscedasticity—a condition where the variance of the errors or residuals remains constant across all levels of the independent variable(s).
Significance of Homoscedasticity
Why does homoscedasticity matter? Its significance lies in its implications for the validity of statistical analyses, particularly regression analysis. When homoscedasticity is violated, it can lead to biased estimates of parameters, inflated or deflated standard errors, and inaccurate hypothesis testing results. In essence, it undermines the reliability of statistical inferences drawn from the data.
Detecting Homoscedasticity
How do we discern whether our data conforms to the assumption of homoscedasticity? There exist various methods and diagnostic tools to assess the presence or absence of homoscedasticity. Visual inspection of residual plots, such as scatterplots of residuals against fitted values or independent variables, can provide valuable insights into the distribution of errors.
Moreover, formal statistical tests, such as the Breusch-Pagan test or White test, can be employed to evaluate the homogeneity of variances across different groups or levels of the independent variable(s). These tests scrutinize the relationship between the squared residuals and the independent variables, thereby gauging the presence of heteroscedasticity.
Implications of Heteroscedasticity
What happens when homoscedasticity is violated? Enter heteroscedasticity, the antithesis of homogeneity in variance. Heteroscedasticity occurs when the spread of residuals systematically varies across different levels of the independent variable(s). This violation of the homoscedasticity assumption can have profound consequences for statistical analyses, rendering regression coefficients inefficient and hypothesis tests unreliable.
Mitigating Heteroscedasticity
Fear not, for there exist remedies to combat the scourge of heteroscedasticity. One approach involves transforming the dependent variable or the independent variable(s) to stabilize the variance of the residuals. Common transformations include logarithmic, square root, or reciprocal transformations, which can help achieve a more uniform spread of residuals.
Additionally, robust standard errors and alternative estimation methods, such as weighted least squares (WLS) or generalized least squares (GLS), can be employed to mitigate the impact of heteroscedasticity on regression analyses. These techniques adjust for the unequal variances of residuals, thereby yielding more reliable parameter estimates and inferential results.
Conclusion
Homoscedasticity, though veiled in statistical jargon, embodies a simple yet crucial concept in the realm of data analysis. It underscores the assumption of equal variances across different levels of independent variables, serving as a cornerstone for robust statistical inference. By understanding the implications of homoscedasticity and its counterpart, heteroscedasticity, analysts can navigate the complexities of regression analysis with clarity and confidence. As we continue to explore the vast expanse of statistical methodologies, let us heed the whispers of homoscedasticity, guiding us towards more accurate and reliable insights from our data.