Unveiling the Mysteries of Effect Size for Chi-Square Analysis
Effect Size for Chi-Square: A Fundamental Exploration
In the vast expanse of statistical analyses, where numbers dance and patterns emerge, one often encounters the enigmatic concept of effect size. Among the myriad statistical tools available, the Chi-Square test stands as a stalwart, especially when dealing with categorical data. Yet, nestled within this statistical juggernaut lies a subtler metric — the effect size for Chi-Square. This article aims to illuminate this intricate topic, unraveling its significance, interpretation, and practical implications.
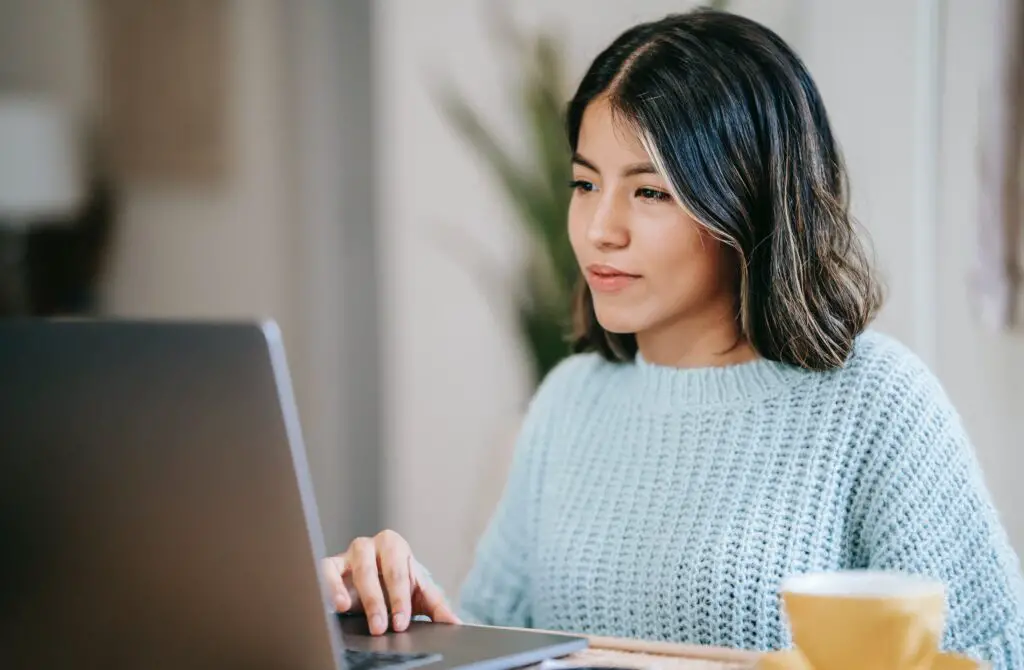
Effect Size for Chi-Square:
What is it?
Effect size, in its essence, serves as a beacon amidst the sea of p-values and statistical significance. It transcends the binary realm of ‘significant’ or ‘not significant,’ providing a quantitative measure of the magnitude of an observed effect. When it comes to Chi-Square analysis, the effect size offers a nuanced understanding beyond mere statistical significance.
In the realm of Chi-Square, the most common effect size measures include Cramer’s V, Phi, and Odds Ratio. Each serves a unique purpose, tailored to the nuances of the data and the research question at hand.
Cramer’s V:
Unveiling the Strength of Association
Cramer’s V, named in homage to the esteemed statistician Harald Cramer, stands as a testament to the strength of association between categorical variables. It operates on a scale from 0 to 1, where 0 denotes no association, and 1 signifies a perfect association. The interpretation of Cramer’s V varies with context, but generally, values closer to 1 indicate a stronger association, while values nearer to 0 suggest a weaker connection.
In the domain of social sciences, for instance, Cramer’s V can shed light on the relationship between variables like gender and political affiliation or educational attainment. A high Cramer’s V value in such scenarios would imply a robust association, guiding researchers towards deeper insights into societal dynamics.
Phi Coefficient:
Unearthing Binary Relations
As a sibling to Cramer’s V, the Phi coefficient specializes in uncovering associations within 2×2 contingency tables. Its simplicity belies its utility, offering a straightforward measure of association between two dichotomous variables. Ranging from -1 to 1, Phi embodies the polarity of association — negative values denote inverse relationships, while positive values signify direct associations.
Consider a classic example: the relationship between smoking and lung cancer. Here, the Phi coefficient could elucidate the strength and direction of this critical association, empowering public health initiatives and policy decisions.
Odds Ratio:
Gauging the Odds in Contingency
In the intricate tapestry of Chi-Square analysis, the Odds Ratio emerges as a powerful tool for understanding the odds of an event occurring across different levels of a categorical variable. Often employed in epidemiology and clinical research, the Odds Ratio transcends the confines of statistical significance, offering insights into the real-world implications of categorical associations.
Imagine a clinical trial evaluating the efficacy of a new drug. By calculating the Odds Ratio, researchers can discern not only the statistical significance of treatment outcomes but also the practical implications for patient care and medical decision-making.
Effect Size for Chi-Square:
A Synthesis of Insight
Effect size for Chi-Square analysis transcends the binary confines of statistical significance, offering a nuanced understanding of categorical associations. Whether through Cramer’s V, Phi, or Odds Ratio, researchers gain a deeper appreciation for the magnitude and direction of these associations, guiding decision-making and advancing scientific inquiry.
In the labyrinth of statistical analyses, where significance tests reign supreme, effect size emerges as a guiding light, illuminating the path towards substantive understanding and meaningful interpretation. So, let us embrace the intricacies of effect size for Chi-Square analysis, for within its depths lie the keys to unlocking the mysteries of categorical relationships.