Deciphering Data:
Understanding Descriptive vs. Inferential Statistics
In the labyrinth of numbers and figures that populate our world, there exists a profound duality: the realms of descriptive and inferential statistics. They are the twin pillars upon which the edifice of data analysis rests, each with its distinct purpose and method. To embark on this journey of comprehension, let us first illuminate the essence of these statistical paradigms.
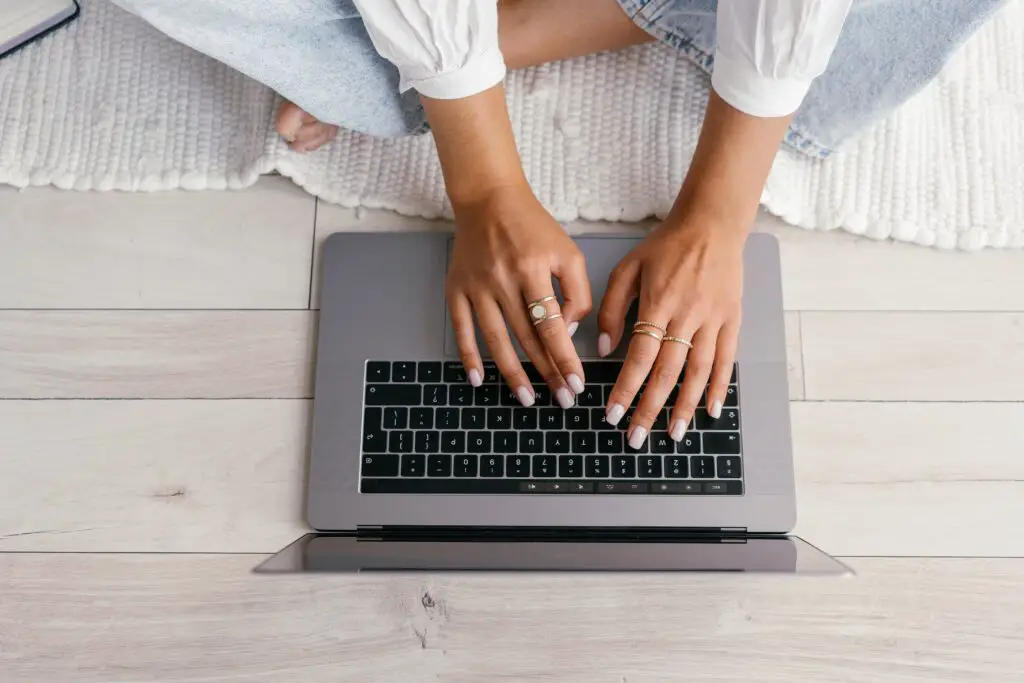
Unveiling the Statistical Tapestry
In the grand tapestry of data analysis, statistics serve as the master weavers, unraveling the intricate patterns hidden within raw information. At the heart of this endeavor lie two fundamental approaches: descriptive and inferential statistics. While both share the common goal of unraveling the mysteries of data, their methodologies diverge, each offering unique insights into the world of numbers.
Descriptive vs. Inferential:
A Dichotomy Defined
At its core, the distinction between descriptive and inferential statistics lies in their primary objectives. Descriptive statistics seek to summarize and present data in a meaningful and comprehensible manner, offering insights into its central tendencies, variability, and distribution. Conversely, inferential statistics venture beyond the realm of observed data, delving into the realm of probability to draw conclusions and make predictions about populations based on sample data.
Descriptive Statistics:
Painting a Portrait of Data
Imagine data as a canvas awaiting the strokes of a skilled artist, poised to unveil its hidden truths. Descriptive statistics serve as the brush, delicately capturing the essence of the data and rendering it into a coherent narrative. Through measures such as mean, median, mode, and standard deviation, descriptive statistics offer a snapshot of the central tendencies and variability inherent within a dataset. Whether charting the rise and fall of stock prices or summarizing survey responses, descriptive statistics provide the foundational framework upon which further analysis can be built.
Inferential Statistics:
Illuminating the Unknown
As the veil of uncertainty shrouds the landscape of data, inferential statistics emerge as the torchbearers, guiding us through the shadows of conjecture and speculation. Drawing upon the principles of probability theory, inferential statistics enable us to extrapolate insights from sample data to make inferences about larger populations. Through techniques such as hypothesis testing and confidence intervals, inferential statistics empower analysts to discern patterns, uncover relationships, and make informed decisions in the face of uncertainty. Whether predicting the outcome of an election or assessing the effectiveness of a new medical treatment, inferential statistics offer a lens through which the unknown becomes knowable.
Navigating the Statistical Terrain:
Practical Applications
In the realm of practical application, descriptive and inferential statistics find myriad uses across diverse domains. Descriptive statistics find utility in summarizing data, identifying outliers, and highlighting trends, serving as the cornerstone of exploratory data analysis. From market research to academic studies, descriptive statistics provide researchers and decision-makers with valuable insights into the nature of their data.
Conversely, inferential statistics empower analysts to move beyond mere observation, unlocking the predictive potential of data through hypothesis testing and regression analysis. In fields as varied as economics, sociology, and epidemiology, inferential statistics play a pivotal role in shaping policy decisions, driving scientific inquiry, and unraveling the complexities of the human experience.
Conclusion
In the grand tapestry of data analysis, descriptive and inferential statistics stand as twin pillars, each offering a unique perspective on the mysteries of data. While descriptive statistics provide a window into the inherent characteristics of a dataset, inferential statistics enable us to peer beyond the veil of uncertainty and draw meaningful conclusions about the world at large. Together, they form the bedrock upon which the edifice of modern data science rests, guiding us through the labyrinth of numbers and leading us towards a deeper understanding of the world around us.