Unraveling the Mysteries of the Chi-Square Test
In the realm of statistics, where numbers dance to unveil truths hidden within data, the Chi-square test stands as a stalwart sentinel. Its name may evoke images of ancient Chinese philosophy or arcane mathematical rituals, but fear not, for it is a tool crafted to bring clarity to the foggy realms of uncertainty. In this journey through the labyrinth of statistical analysis, we shall traverse the landscapes of hypothesis testing, contingency tables, and categorical data, guided by the beacon of the Chi-square test.
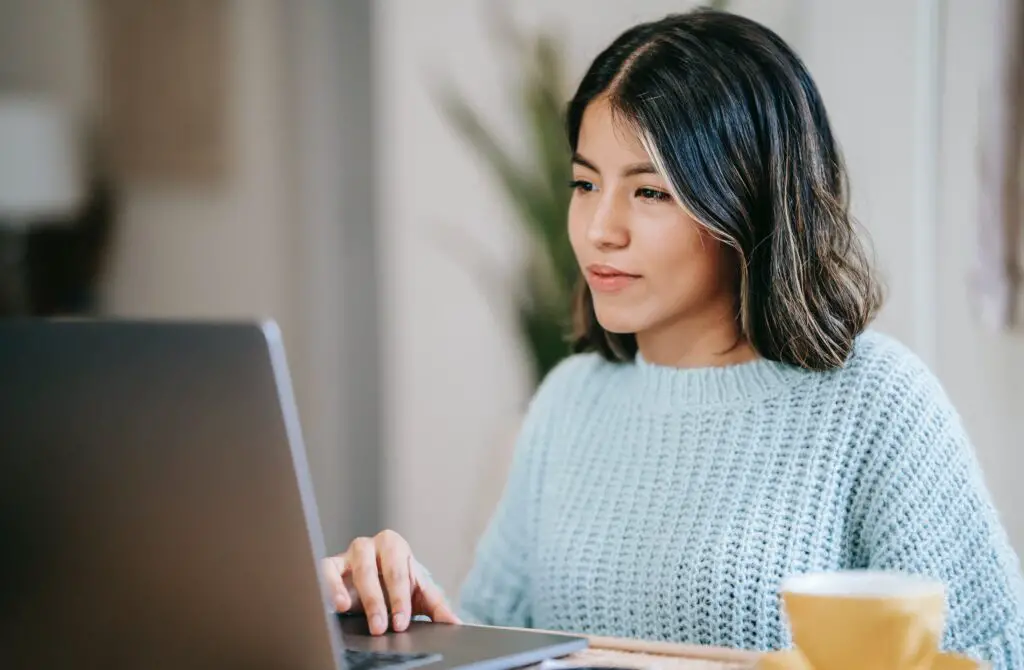
Chi-Square Test
In the dimly lit corridors of statistical analysis, the Chi-square test emerges as a shining beacon, illuminating the path towards understanding the relationships between categorical variables. At its core, this test seeks to answer a fundamental question: Are the observed frequencies of different categories significantly different from what we would expect by chance alone?
Chi-Square Test: Unveiling the Mechanics
To wield the Chi-square test with finesse, one must first comprehend its inner workings. Picture a grand banquet table adorned with an array of sumptuous dishes, each representing a different category. Now, imagine a group of guests arriving, each with their preferences and biases. The Chi-square test endeavors to determine whether the distribution of guests around this table is merely a result of chance or if there’s a deeper pattern at play.
Chi-Square Test in Action
Imagine a scenario where a researcher seeks to investigate whether there’s a relationship between gender and political affiliation. They gather a cohort of individuals and record their genders and respective political leanings. Armed with this data, the researcher constructs a contingency table, a tabular arrangement where rows represent genders and columns represent political affiliations. The Chi-square test then steps onto the stage, scrutinizing the observed frequencies in each cell of this table and comparing them to the frequencies we would expect if there were no relationship between gender and political affiliation.
Interpreting the Results
Once the Chi-square test has completed its calculations, it bestows upon us a p-value, a numerical representation of the likelihood that the observed differences in frequencies are purely due to chance. Should this p-value be sufficiently low, we’re granted the boon of statistical significance, indicating that the observed data deviates from what we’d expect under the assumption of no relationship between the variables.
Chi-Square Test: A Versatile Tool
But lo, the Chi-square test is not confined to the narrow corridors of gender and political affiliation alone. Nay, its reach extends far and wide, embracing diverse fields of inquiry with open arms. From genetics to sociology, from market research to epidemiology, wherever categorical variables dance in the tapestry of data, the Chi-square test stands ready to illuminate the patterns woven within.
Common Pitfalls and Limitations
Yet, even as we bask in the glow of the Chi-square test’s enlightenment, we must tread with caution, for pitfalls lie in wait for the unwary. Small sample sizes may render our results unreliable, while violations of assumptions, such as the independence of observations or the adequacy of expected cell frequencies, can cast shadows of doubt upon our conclusions. Thus, we must approach the Chi-square test with a discerning eye, mindful of its strengths and limitations alike.
Conclusion
In the realm of statistical analysis, where uncertainty reigns supreme, the Chi-square test emerges as a steadfast ally, guiding us through the tangled thickets of categorical data with grace and precision. Armed with its insights, we traverse the landscapes of inquiry, uncovering the hidden patterns that shape our understanding of the world. So let us raise our glasses to the Chi-square test, a beacon of illumination in the foggy realms of uncertainty.