Unveiling the Intricacies of Categorical Variables
In the vast landscape of data science and statistics, certain terms hold a mystique that piques curiosity and prompts deeper exploration. Among these, the enigmatic “categorical variable” stands as a cornerstone concept, wielding profound significance in the realms of analysis, modeling, and understanding complex datasets.
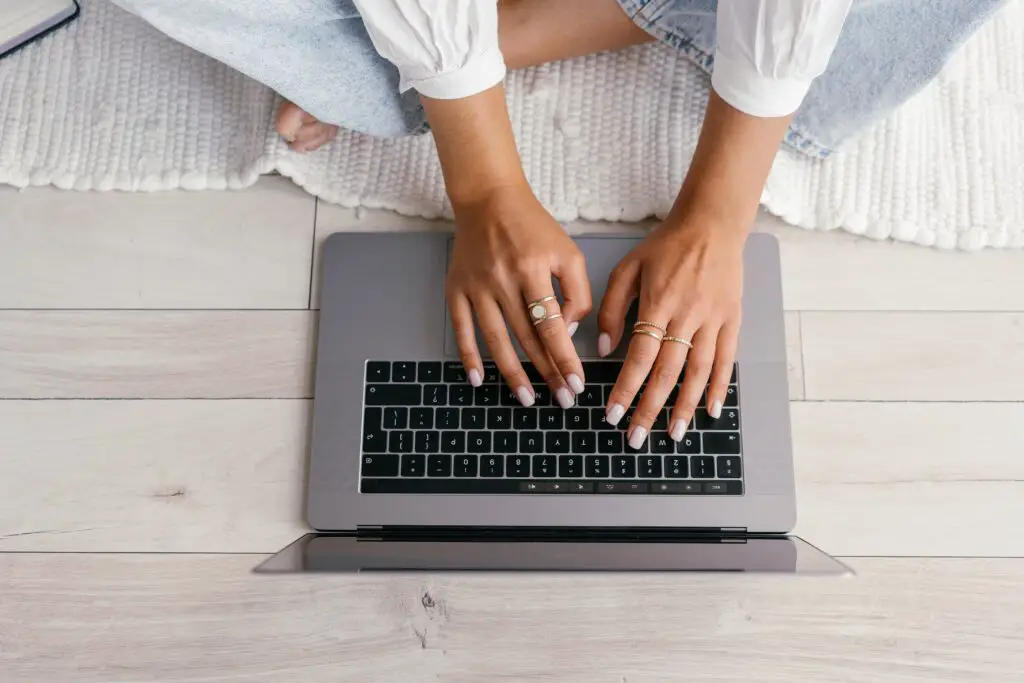
Categorical Variable:
Deciphering the Essence
At its essence, a categorical variable embodies a fundamental aspect of data classification, delineating distinct groups or categories within a dataset. These categories are not inherently ordered in a quantitative sense but rather represent qualitative distinctions that capture diverse characteristics or attributes.
Categorizing the Uncategorized
The crux of a categorical variable lies in its ability to classify data into discrete groups based on specific attributes or characteristics. Imagine a dataset encompassing information about individuals’ professions—each entry could fall into distinct categories such as “doctor,” “teacher,” “engineer,” or “artist.” Here, the variable “profession” serves as a categorical entity, organizing the data into meaningful groups that facilitate analysis and interpretation.
Diving Deeper: Types of Categorical Variables
Within the realm of categorical variables, nuances abound, with various subtypes enriching the analytical landscape. One common classification distinguishes between nominal and ordinal variables, each imbued with unique characteristics and analytical implications.
Nominal Variables: Embracing Unordered Diversity
Nominal variables encompass categories devoid of any inherent order or hierarchy. They represent qualitative distinctions that lack a prescribed sequence. Returning to the example of professions, nominal variables would encompass classifications such as “doctor,” “teacher,” or “artist,” where no inherent ranking exists among the categories. Instead, each category holds equal footing within the variable, serving as distinct labels for different groups.
Ordinal Variables: Grasping the Essence of Order
In contrast, ordinal variables introduce a semblance of order or hierarchy among categories, albeit in a qualitative rather than quantitative sense. These variables imbue categories with a structured relationship, allowing for distinctions such as “low,” “medium,” and “high” or “small,” “medium,” and “large.” Returning to our professional dataset, an ordinal variable might categorize individuals based on their level of experience—ranging from “novice” to “intermediate” to “expert.” Here, while the categories possess distinct labels, they also exhibit a discernible order reflecting increasing levels of expertise or proficiency.
Navigating the Analytical Terrain: Applications of Categorical Variables
The ubiquitous presence of categorical variables permeates diverse domains, fueling a myriad of analytical endeavors and applications. From market segmentation and customer profiling to medical diagnosis and social research, these variables serve as indispensable tools for unraveling complex patterns, discerning relationships, and extracting actionable insights from data.
Unveiling Patterns Through Visualization
Visual representation stands as a powerful ally in the exploration and interpretation of categorical variables. Bar charts, pie charts, and stacked bar plots emerge as stalwart companions, offering intuitive depictions of categorical distributions, proportions, and relationships. Through vibrant visualizations, intricate patterns and trends embedded within categorical data come to life, fostering deeper comprehension and informed decision-making.
Embracing the Complexity: Challenges and Considerations
While categorical variables offer a gateway to unraveling the intricacies of data, they also present challenges and considerations worthy of acknowledgment. Chief among these is the phenomenon of multicollinearity, wherein correlations between categorical variables can confound analyses and distort results. Additionally, the curse of dimensionality looms large, particularly in datasets featuring a plethora of categorical attributes, necessitating judicious feature selection and dimensionality reduction techniques to mitigate computational burdens and enhance model performance.
The Quest for Insight: Leveraging Categorical Variables in Machine Learning
In the ever-evolving landscape of machine learning, categorical variables assume a pivotal role, serving as indispensable features in predictive modeling and algorithmic frameworks. Techniques such as one-hot encoding and label encoding empower practitioners to harness the predictive potential of categorical attributes, seamlessly integrating them into sophisticated machine learning pipelines. Through strategic feature engineering and adept model selection, practitioners unlock the latent insights harbored within categorical variables, paving the way for enhanced predictive accuracy and actionable intelligence.
Evolving Perspectives: Beyond the Binary
As the boundaries of data science continue to expand, so too does our conception of categorical variables. Beyond the traditional dichotomy of nominal and ordinal distinctions, emerging paradigms such as hierarchical categorization and fuzzy logic usher in new vistas of analytical possibility, challenging conventional notions and catalyzing innovation in the pursuit of knowledge and understanding.
Conclusion
In the tapestry of data science, categorical variables stand as pillars of classification, imbuing datasets with structure, meaning, and insight. From their humble origins as labels and descriptors to their pivotal roles in predictive modeling and machine learning, these variables embody the essence of qualitative distinction, shaping our understanding of the intricate patterns and relationships that define our world. As we traverse the analytical terrain, let us embrace the diversity and richness of categorical variables, harnessing their power to illuminate the hidden truths and unlock the boundless potential of data-driven discovery.