Exploring the Nuances of Statistical Analysis:
Anova vs. T-Test
In the intricate realm of statistics, where numbers dance to uncover hidden truths and patterns, two powerful tools stand out: the Analysis of Variance (ANOVA) and the t-test. These statistical methods serve as guiding lights for researchers, illuminating pathways to sound conclusions and robust insights. But what sets them apart? Why choose one over the other? Join me on a journey through the landscapes of ANOVA and t-tests as we unravel their intricacies and unveil their unique roles in the realm of statistical analysis.
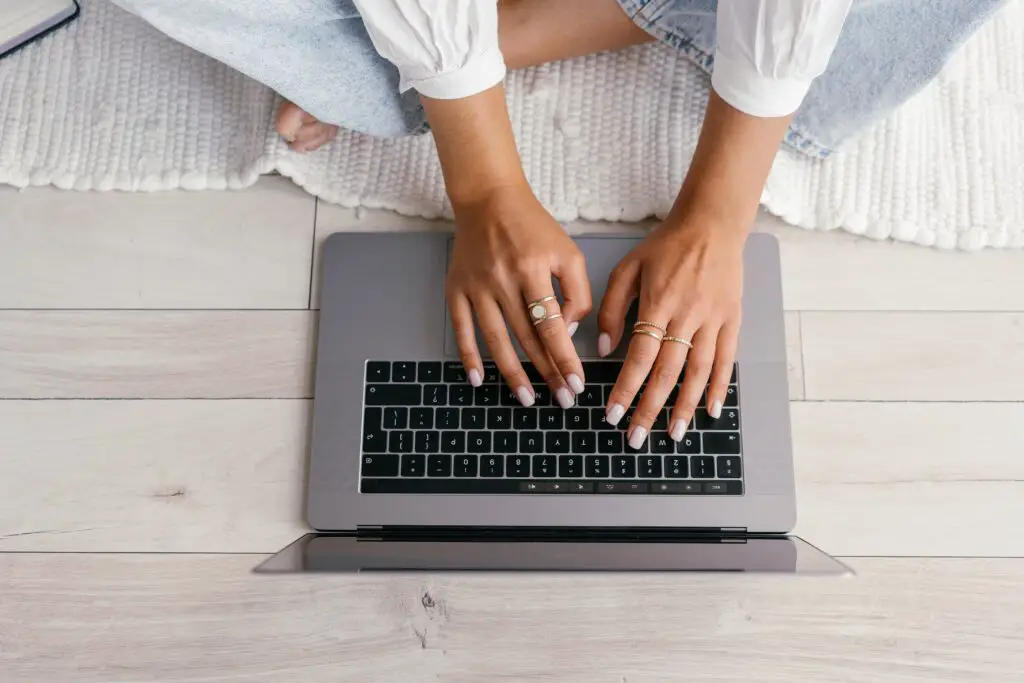
Unveiling the Tale of Two Statistical Titans
At the heart of scientific inquiry lies the quest for understanding, driven by the pursuit of answers to questions both profound and practical. In this pursuit, researchers rely on statistical methods to sift through data, discern patterns, and draw meaningful conclusions. Among the myriad tools in the statistical arsenal, ANOVA and t-tests emerge as stalwart companions, each wielding its own strengths and subtleties.
ANOVA:
Illuminating the Power of Variation
Analysis of Variance, or ANOVA, stands as a beacon of insight into the realm of variation. Its roots delve deep into the understanding of differences between groups, shedding light on the nuanced interplay of factors within a dataset. ANOVA excels in scenarios where multiple groups are compared simultaneously, offering a comprehensive lens through which to assess the significance of these group disparities.
T-Test: Navigating the Terrain of Means
Meanwhile, the t-test emerges as a versatile tool, adept at navigating the terrain of means. Whether exploring the difference between two means or delving into the subtleties of paired observations, the t-test serves as a trusty guide, illuminating pathways to inference with elegance and precision. Its simplicity belies its power, making it a cornerstone of statistical analysis across diverse fields.
5 Statistics Case Studies:
A Dive into Practical Applications
Case Study 1: Comparing Exam Scores
Question: You are a teacher interested in comparing the exam scores of students who received different types of instruction: traditional lectures, online tutorials, and interactive workshops. Which statistical method would best suit your analysis?
Answer: ANOVA would be the ideal choice here. With multiple groups to compare (traditional lectures, online tutorials, and interactive workshops), ANOVA allows for a comprehensive assessment of variance between these groups, providing valuable insights into the efficacy of different instructional methods.
Case Study 2: Assessing Drug Efficacy
Question: A pharmaceutical company is conducting a study to assess the efficacy of a new drug compared to a placebo. Which statistical method would be most appropriate for analyzing the results?
Answer: In this scenario, a two-sample t-test would be the method of choice. With a straightforward comparison between two independent groups (those receiving the new drug versus those receiving a placebo), the t-test offers a robust means of determining whether the observed differences in outcomes are statistically significant.
Case Study 3: Examining Customer Satisfaction
Question: A retail chain wants to evaluate customer satisfaction levels across its various store locations. How should they approach the statistical analysis of customer feedback data?
Answer: Given the categorical nature of the data (different store locations), ANOVA would be the preferred method for this analysis. By examining variation in customer satisfaction scores across multiple groups (store locations), ANOVA enables the identification of any significant differences that may exist between these groups.
Case Study 4: Investigating Employee Productivity
Question: A human resources department seeks to investigate whether flexible work arrangements lead to differences in employee productivity. What statistical approach should they adopt for their analysis?
Answer: Here, a paired t-test would be most appropriate. By comparing the productivity levels of individual employees before and after the implementation of flexible work arrangements, a paired t-test allows for the assessment of within-subject differences while controlling for individual variability, providing valuable insights into the impact of flexible work policies.
Case Study 5: Evaluating Marketing Strategies
Question: A marketing team wants to assess the effectiveness of three different advertising strategies in driving sales. How can they determine which strategy yields the best results?
Answer: Once again, ANOVA emerges as the statistical method of choice. By comparing sales outcomes across multiple advertising strategies simultaneously, ANOVA enables the identification of any significant differences in effectiveness, empowering the marketing team to make data-driven decisions regarding resource allocation and strategy refinement.
In the vast landscape of statistical analysis, ANOVA and t-tests stand as pillars of insight, offering distinct yet complementary approaches to unraveling the mysteries hidden within data. From the exploration of variation to the comparison of means, these tools pave the way for discovery, guiding researchers toward deeper understanding and informed decision-making. As we continue to navigate the ever-evolving terrain of data analysis, let us embrace the versatility and power of ANOVA and t-tests, harnessing their potential to illuminate the path forward in our quest for knowledge.