The Intricacies of Standard Deviations:
Unraveling the Mystery
In the grand tapestry of statistics, the standard deviation stands as a crucial thread, weaving through the fabric of data analysis and inference. It’s a measure that encapsulates the dispersion or variability of a dataset, offering invaluable insights into the spread of values around the mean. Yet, amidst its mathematical elegance, a question occasionally arises: can standard deviations be negative?
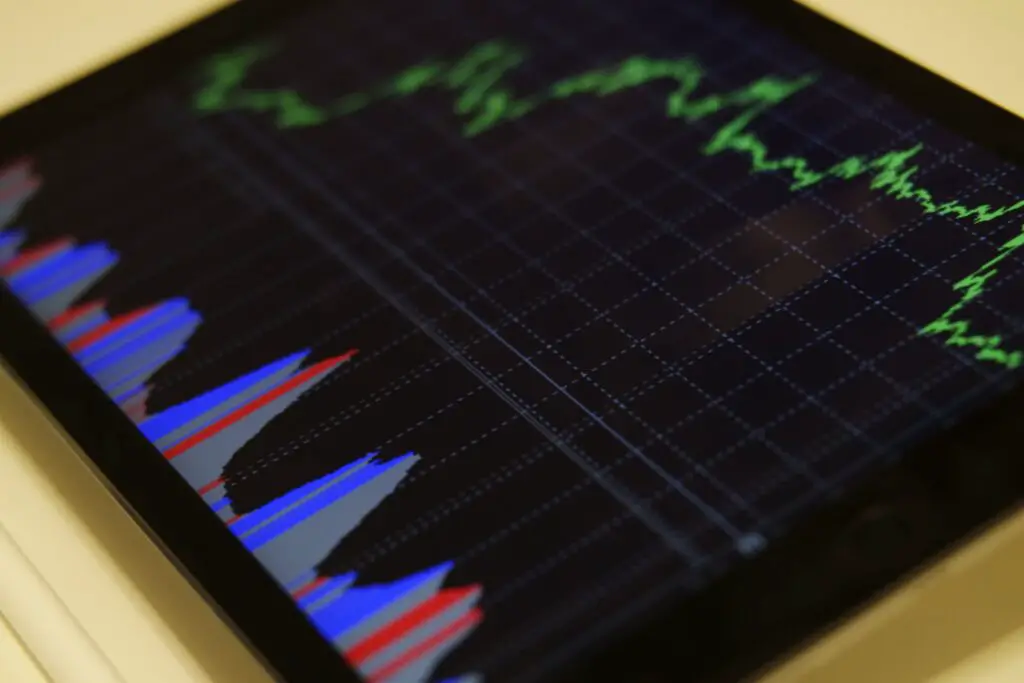
Can Standard Deviations be Negative?
In the realm of statistical analysis, the concept of a negative standard deviation might seem as incongruous as a square circle or a silent symphony. After all, how could a measure of dispersion, ostensibly rooted in the positivity of numbers, ever dip into the realm of negativity?
The answer, unequivocally, is no. Standard deviations cannot be negative.
At its core, the standard deviation is a measure of variability that quantifies how much individual data points deviate from the mean of a dataset. By squaring the deviations from the mean, summing them up, dividing by the number of observations, and then taking the square root, statisticians arrive at this quintessential metric. However, this process ensures that the standard deviation emerges as a non-negative value.
Consider the mathematical steps involved: squaring deviations eliminates negative signs, ensuring that all values are positive before summing them. Consequently, when this squared sum is divided by the number of observations and the square root is taken, the result can only be non-negative. This intrinsic property of the standard deviation renders the notion of a negative value null and void.
But let us delve deeper into the rationale behind this mathematical constraint.
Understanding the Mathematics Behind Standard Deviation
To comprehend why standard deviations cannot be negative, one must first grasp the mathematical underpinnings of this statistical measure. At its essence, the standard deviation encapsulates the dispersion of data points around the mean, offering a succinct summary of the variability inherent within a dataset.
The calculation of the standard deviation involves several sequential steps, each designed to distill the essence of variability while preserving the integrity of mathematical operations. Initially, the deviations of individual data points from the mean are squared, transforming any negative deviations into positive quantities. This step not only harmonizes the disparate directions of deviation but also accentuates the magnitude of deviations, thereby magnifying the influence of outliers.
Following this, the squared deviations are summed together, amalgamating the dispersed energies of data points into a cohesive whole. This cumulative sum represents the collective variability embedded within the dataset, a testament to the diverse nature of empirical observations.
Subsequently, the squared sum is divided by the total number of observations, yielding an average measure of variability that is reflective of the dataset’s intrinsic characteristics. By normalizing the sum of squared deviations, statisticians obtain a nuanced perspective on the dispersion of data points, free from the shackles of scale or magnitude.
Finally, the square root of this quotient is taken, culminating in the emergence of the standard deviation—a definitive metric that encapsulates the essence of variability within the dataset. This final step ensures that the standard deviation maintains dimensional consistency with the original data, preserving the interpretability of statistical analyses.
Throughout this meticulous process, one overarching principle remains steadfast: the preservation of positivity. By squaring deviations and subsequently taking the square root, statisticians ensure that the standard deviation emerges as a non-negative quantity, in alignment with the fundamental tenets of mathematics.
Implications and Applications
The impossibility of negative standard deviations has profound implications across various domains of inquiry, ranging from scientific research to financial analysis. In the scientific arena, standard deviations serve as a compass guiding researchers through the labyrinth of empirical data, illuminating the pathways of discovery with insights into the variability of observations.
In fields such as finance and economics, standard deviations assume an even greater significance, offering a beacon of stability amidst the tumultuous seas of market volatility. By quantifying the dispersion of asset returns or price fluctuations, standard deviations empower investors and analysts to navigate the capricious currents of financial markets with prudence and foresight.
Moreover, the impossibility of negative standard deviations underscores the importance of rigorous mathematical reasoning in statistical analyses. As guardians of empirical truth, statisticians and data scientists must remain vigilant against the specter of mathematical fallacies, ensuring that their interpretations remain grounded in the immutable laws of logic and reason.
Conclusion
In the vast expanse of statistical analysis, the standard deviation stands as a beacon of clarity amidst the fog of uncertainty—a measure that distills the essence of variability into a single, concise metric. While the question of negative standard deviations may occasionally flicker in the recesses of the mind, a thorough examination of mathematical principles swiftly dispels any semblance of doubt.
Through the lens of mathematics, we perceive the intrinsic impossibility of negative standard deviations—a testament to the elegance and precision of statistical reasoning. In embracing this mathematical truth, we reaffirm our commitment to empirical inquiry and logical rigor, charting a course towards a deeper understanding of the intricate tapestry of data.